Publications
A collaboration with the FDA: new insights using real-world evidence
This latest study applies our method of assessing disease progression in real-world cancer datasets to a large cohort of patients with advanced NSCLC treated with PD-1/PD-L1 inhibitors...
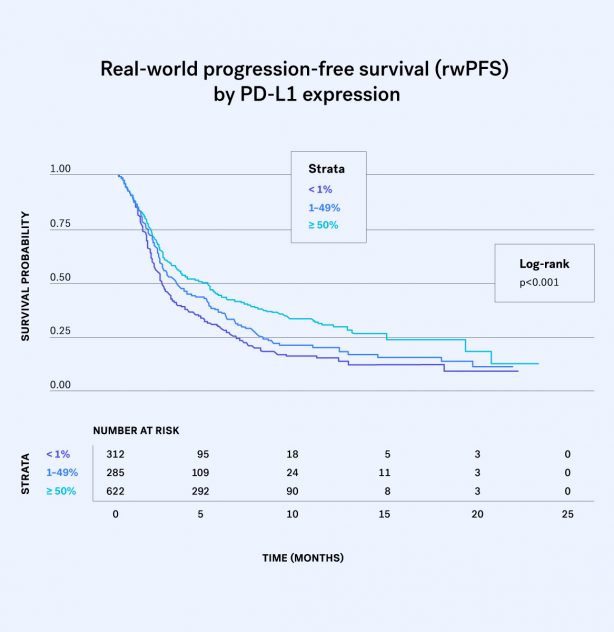
CDISC Standards: A Crucial Requirement for FDA Submissions in Clinical Research
Miscellaneous
|The FDA’s Stance on CDISC Standards
The FDA has been working closely with CDISC since its inception to develop and implement data standards that facilitate the regulatory review process. As a result, CDISC standards have become mandatory for all clinical studies submitted to the FDA since December 2016. This requirement underscores the importance of CDISC standards in the pharmaceutical industry and their role in expediting the drug approval process.Key CDISC Standards Required by the FDA
Since December 17, 2016, the FDA has required the use of CDISC standards for new drug applications (NDAs), biologics license applications (BLAs), and abbreviated new drug applications (ANDAs) involving studies initiated after December 17, 2014. This requirement is outlined in the FDA’s Study Data Standards Resources, which include:- Study Data Technical Conformance Guide (TCG) - A resource that provides technical recommendations for preparing and submitting standardized study data to the FDA
- Data Standards Catalog – A resource that specifies which versions of data standards and terminologies are supported by the FDA for use in regulatory submissions
- Study Data Tabulation Model (SDTM) - A standard for clinical data in tabular form, designed to facilitate data aggregation and sharing
- Analysis Data Model (ADaM) - A standard for analysis-ready datasets derived from SDTM
- CDASH (Clinical Data Acquisition Standards Harmonization) - A standard for the capture of clinical data, optimized for ease of collection, data quality, and subsequent transformation into SDTM format
- SEND (Standard for Exchange of Nonclinical Data) - A standard for preclinical data, such as toxicology studies in animals
Benefits of CDISC Standards in Clinical Research
The implementation of CDISC standards offers numerous advantages to the pharmaceutical and biotech industries:- Increased efficiency
- Enhanced data quality
- Facilitated data sharing
Implementing CDISC Standards: Challenges and Solutions
Adopting CDISC standards can present challenges for organizations, particularly those with established data management processes:- High initial costs
- Complexity of data transformation
- Keeping up with updates
- Early planning
- Leveraging technology
- Continuous training
- Collaboration with experts
The Future of CDISC Standards and FDA Requirements
As the field of clinical research continues to evolve, especially with the potentially transformational role of AI in drug development, CDISC standards are likely to adapt and expand. The FDA has demonstrated an ongoing commitment to data standardization, as evidenced by its Data Standards Strategy for fiscal years 2023–2027. This strategy emphasizes the continued importance of CDISC standards in regulatory submissions and highlights areas for future development. Some potential areas of growth for CDISC standards include:- Integration with real-world data sources
- Adaptation to emerging therapeutic areas, such as gene and cell therapies
- Enhanced support for patient-reported outcomes and wearable device data
Unlocking Data Potential: Understanding FAIR vs Open Data in Life Sciences
Miscellaneous
|Understanding FAIR Data
FAIR data is a set of guiding principles for scientific data management and stewardship. Their aim is to optimize data management to enable machines and humans to effectively find, access, and utilize data. The acronym FAIR stands for Findable, Accessible, Interoperable, and Reusable. These principles were developed to support the reusability of digital assets, addressing the increasing volume and complexity of data generated in modern research.- Findable
- Assigning unique and persistent identifiers to datasets and entities
- Providing rich metadata that describes the data in detail
- Registering or indexing the data in a searchable resource
- The data is retrievable by its identifier using a standardized protocol
- The protocol is open, free, and universally implementable
- The metadata remains accessible even when the data is no longer available
- Using a shared and broadly applicable language for knowledge representation
- Utilizing standard vocabularies that follow FAIR principles
- Including qualified references to other data
- Having clear and accessible data usage licenses
- Providing detailed data provenance information
- Meeting domain-relevant community standards
Benefits of FAIR Data in Life Sciences
Pharmaceutical and biotech companies rely heavily on FAIR data to:- Streamline data sharing across international research teams
- Reduce duplication of effort by making datasets reusable
- Facilitate machine learning and artificial intelligence in drug discovery
- Ensure regulatory compliance by maintaining detailed data provenance
Open Data: A Different Approach
Open data, on the other hand, focuses on making data freely available to everyone to use and republish without restrictions. Open data is rooted in the ideas of transparency, collaboration, and unrestricted sharing to promote innovation and societal benefit. The key characteristics of open data include:- Availability and access - The data must be freely accessible to all, preferably by downloading over the internet without paywalls or complex permissions, at no more than a reasonable reproduction cost.
- Reuse and redistribution - There are no legal or technical restrictions on how the data can be utilized. The data must be provided under terms that permit reuse and redistribution, including the intermixing with other datasets.
- Transparency - Open data emphasizes full transparency in its collection, processing, and sharing.
Benefits of Open Data in Life Sciences
In the life sciences sector, open data has been instrumental in:- Accelerating research by providing unrestricted access to key datasets such as The Cancer Genome Atlas (TCGA)
- Enhancing reproducibility by making data available for validation studies
- Promoting public trust in science by ensuring transparency in research findings
Key Differences between FAIR and Open Data
While FAIR data and open data share some common goals, they differ in several important aspects:- Accessibility requirements
- Focus on machine readability
- Metadata and documentation
- Interoperability standards
Aspect | FAIR Data | Open Data |
---|---|---|
Accessibility | Can be open or restricted based on use case | Always open to all |
Focus | Ensures data is machine-readable and reusable | Promotes unrestricted sharing and transparency |
Licensing | Varies—can include access restrictions | Typically utilizes open licenses like Creative Commons |
Primary Users | Designed for researchers, institutions, and machines | Designed for public and scientific communities |
Application | Ideal for structured data integration in R&D | Ideal for democratizing access to large datasets |
Implications for Life Sciences Industries
The distinction between FAIR and open data has significant implications for pharmaceutical, biotech, and healthcare industries:- Data sharing in collaborative research
- Clinical trial data management
- Genomics and bioinformatics
- Regulatory compliance
Integrating FAIR and Open Data Strategies
In many cases, life sciences organizations find value in combining FAIR and open data principles. For example:- A biotech company might use FAIR principles to manage its proprietary datasets while contributing anonymized, aggregated data to open repositories for public benefit.
- Government-funded research institutions often follow FAIR principles internally and publish open data externally to comply with transparency mandates.
The Future of Data Management in Life Sciences
As the life sciences continue to generate increasingly complex and voluminous data, the principles of FAIR data are likely to become even more critical. While open data will continue to play an important role, particularly in publicly funded research, the nuanced approach of FAIR data is better suited to the complex needs of the pharmaceutical and biotech industries. By adopting FAIR data principles, companies can:- Enhance the value of their data assets
- Improve collaboration and data sharing
- Accelerate the pace of discovery and innovation
- Ensure better compliance with regulatory requirements
- Increase the reproducibility of research findings
Role of Data Curation and Bioinformatics Services
Organizations operating in life sciences can overcome these challenges by partnering with specialized bioinformatics service providers like Rancho Biosciences, which offers:- Data curation and governance - Ensuring datasets adhere to FAIR principles while meeting regulatory standards
- Custom workflows and pipelines - Designing interoperable systems for seamless data integration
- Knowledge mining and database building - Extracting actionable insights from both proprietary and open data
The Science of Safety: Understanding Toxicology in Biomedical Research
Miscellaneous
|The Fundamentals of Toxicology
At its core, toxicology is the study of the adverse effects of chemicals or physical agents on living organisms. This discipline encompasses a wide range of research areas, including:- Mechanistic toxicology
- Regulatory toxicology
- Environmental toxicology
- Clinical toxicology
Toxicology in Drug Development
The pharmaceutical industry relies heavily on toxicological studies to ensure the safety of new drugs before they reach clinical trials. This process involves several key stages:- Preclinical safety assessment
- Identify potential target organs for toxicity
- Determine safe starting doses for human trials
- Predict potential side effects
- Establish safety margins between therapeutic and toxic doses
- Toxicokinetics and pharmacokinetics
- Determine the relationship between drug dose and toxic effects
- Identify potential drug-drug interactions
- Optimize dosing regimens to minimize toxicity while maintaining efficacy
- Genotoxicity and carcinogenicity testing
- In vitro mutagenicity assays (e.g., Ames test)
- In vivo chromosomal aberration studies
- Long-term carcinogenicity studies in rodents
Emerging Trends in Toxicology
The field of toxicology is continuously evolving, incorporating new technologies and approaches to enhance safety assessments. Some notable trends include:- Toxicogenomics
- Identify biomarkers of toxicity
- Predict potential adverse effects based on gene expression patterns
- Understand individual susceptibility to specific substances
- 3D cell culture models
- Better prediction of human toxicity compared to traditional 2D cell cultures
- Reduced reliance on animal testing
- Improved understanding of organ-specific toxicity mechanisms
- Artificial intelligence and machine learning
- Rapid screening of large chemical libraries for potential toxicity
- Identification of complex patterns in toxicological data
- Development of more accurate in silico toxicity prediction models
Regulatory Landscape and Toxicology
Toxicological studies are essential for meeting regulatory requirements and ensuring public safety. Key regulatory bodies and guidelines include:- FDA (Food and Drug Administration) - Oversees drug safety in the United States
- EMA (European Medicines Agency) - Regulates medicines in the European Union
- ICH (International Council for Harmonisation) - Provides global guidelines for drug development and safety assessment
- Good Laboratory Practice (GLP) regulations
- Specific guidelines for different types of toxicity studies (e.g., reproductive toxicity, chronic toxicity)
- Requirements for toxicology data submission in regulatory dossiers
Challenges and Future Directions
Despite significant advancements, toxicology in biomedical science faces several challenges:- Translating animal data to humans
- Humanized animal models
- Advanced in vitro systems that better mimic human physiology
- Integrative approaches combining multiple data sources
- Assessing multiple-component toxicity
- Developing models to predict multiple-component toxicity
- Studying additive, synergistic ,and antagonistic interactions between compounds
- Assessing cumulative exposure risks in environmental and occupational settings
- Addressing emerging contaminants
- Develop specialized testing methods for novel substances
- Understand long-term effects of chronic low-dose exposures
- Assess potential environmental impacts of emerging contaminants
Understanding Data Integrity: The Backbone of Biomedical Research
Miscellaneous
|What Is Data Integrity in Biomedical Research?
Data integrity in biomedical research refers to the accuracy, completeness, consistency, and reliability of data throughout its lifecycle. It encompasses the entire process of data collection, storage, analysis, and reporting. In essence, data integrity ensures the information used in research is trustworthy, traceable, and can withstand scrutiny.Core Principles of Data Integrity
The ALCOA+ framework, widely recognized within the scientific community, defines the fundamental principles of data integrity as follows:- Attributable - All data should be traceable to its origin, including who created it and when.
- Legible - Data must be readable and permanently recorded.
- Contemporaneous - Data should be recorded at the time of generation.
- Original - Primary records should be preserved and any copies clearly identified.
- Accurate - Data should be free from errors and reflect actual observations.
- Complete - All data, including metadata, should be present.
- Consistent - Data should align across different sources and versions.
- Enduring - Data should be preserved in a lasting format.
- Available - Data should be readily accessible for review and audit.
The Importance of Data Integrity
- Ensuring research reliability and reproducibility
- Safeguarding patient safety
- Regulatory compliance
Challenges to Data Integrity in Biomedical Research
- Data volume and complexity
- Data heterogeneity
- Human error and bias
- Cybersecurity threats
Best Practices for Ensuring Data Integrity
- Implement robust data management systems
- Audit trails to track all data modifications
- Version control to manage changes over time
- Access controls to prevent unauthorized data manipulation
- Data validation checks to ensure accuracy and completeness
- Standardize data collection and documentation
- Using validated instruments and methods
- Implementing standardized data formats and terminologies
- Maintaining detailed metadata to provide context for the data
- Conduct regular data quality assessments
- Regular data audits to identify and correct errors
- Statistical analyses to detect anomalies or outliers
- Cross-validation of data from different sources
- Invest in training and education
- Offering regular training sessions on data management and integrity
- Fostering a culture of data quality and ethical research practices
- Providing clear guidelines and standard operating procedures
- Leverage advanced technologies
- Artificial intelligence and machine learning for automated data validation and anomaly detection
- Blockchain technology for creating immutable data records
- Natural language processing for extracting structured data from unstructured sources
The Role of Data Management Professionals in Ensuring Data Integrity
Firms that specialize in biomedical data services play a crucial role in helping pharmaceutical, biotech, and research organizations uphold the highest standards of data integrity. Look for a company with a comprehensive suite of services that includes:- Data curation and standardization
- Quality control and validation
- Advanced analytics and data integration
- Custom data management solutions
Navigating the Data Jungle: How Ontology & Taxonomy Shape Biomedical Research
Miscellaneous
|What Is Data Taxonomy?
Definition & Key Characteristics Data taxonomy is a hierarchical classification system that organizes information into categories and subcategories based on shared characteristics. In the context of biomedical research, taxonomies provide a structured way to classify entities such as diseases, drugs, and biological processes. Key characteristics of taxonomies include:- Hierarchical structure
- Clear parent-child relationships
- Comprehensive coverage of a domain
- Drug classification - Organizing pharmaceuticals based on their chemical structure, mechanism of action, or therapeutic use
- Disease classification - Categorizing diseases according to their etiology, affected body systems, or pathological features
- Biological classification - Organizing living organisms based on their evolutionary relationships and shared characteristics
- Simplicity and ease of understanding
- Efficient navigation of large datasets
- Clear categorization for data retrieval
- Rigidity in structure
- Difficulty in representing complex relationships
- Limited ability to capture cross-category connections
What Is Data Ontology?
Definition & Key Characteristics Data ontology is a more complex and flexible approach to data organization. It represents a formal explicit specification of a shared conceptualization within a domain. Unlike taxonomies, ontologies capture not only hierarchical relationships but also other types of associations between concepts. Key characteristics of ontologies include:- Rich relationship types
- Formal logic and rules
- Machine-readable format
- Cross-domain integration capabilities
- Knowledge representation - Capturing complex biomedical concepts and their interrelationships
- Data integration - Facilitating the integration of diverse datasets from multiple sources
- Semantic reasoning - Enabling automated inference and discovery of new knowledge
- Natural language processing - Supporting the extraction of meaningful information from unstructured text
- Ability to represent complex relationships
- Support for automated reasoning and inference
- Flexibility in accommodating new knowledge
- Enhanced interoperability between different data sources
- Higher complexity and steeper learning curve
- Increased resource requirements for development and maintenance
- Potential for inconsistencies in large collaborative ontologies
Key Differences between Taxonomy & Ontology
Although both data ontology and taxonomy aim to organize data, the key differences between them lie largely in their structure, complexity, use cases, and more. Determining whether you’re working with a taxonomy or an ontology can sometimes be challenging, as both systems organize information and some of the same tools may be used for viewing both.Aspect | Data Taxonomy | Data Ontology | ||||
Structure | Hierarchical tree-like structure with clear parent-child relationships | Relational, capturing complex interconnections between entities | ||||
Complexity |
|
|
||||
Flexibility |
|
|
||||
Use Case |
|
|
||||
Industry Example |
|
Understanding gene-disease-drug relationships in genomics research | ||||
Inference Capabilities | Limited to hierarchical inferences | Supports complex reasoning and automated inference | ||||
Interoperability | Generally domain-specific | Facilitates cross-domain integration and knowledge sharing |
Why These Differences Matter in Life Sciences & Biotech
In life sciences and biotech, data isn’t just increasing in volume but also in complexity. The ability to extract meaningful insights from data is critical for drug discovery, patient treatment, and regulatory compliance. Knowing when to use a taxonomy versus an ontology can greatly affect the quality and efficiency of data governance and analysis. For instance, taxonomies can help with organizing large datasets in clinical research, making it easier for teams to categorize patient data, drugs, and treatment outcomes. However, when the goal is to understand how a drug interacts with different biological pathways or to predict patient responses based on genetic profiles, ontologies become essential. By mapping complex relationships, ontologies provide the deep contextual understanding required to drive precision medicine and personalized treatments.Choosing between Taxonomy & Ontology in Biomedical Research
The decision to use a taxonomy or ontology depends on several factors: When to Use Taxonomy- For simple hierarchical classification of well-defined entities
- When rapid development and deployment are priorities
- In scenarios where user-friendly navigation is crucial
- For projects with limited resources or expertise in ontology development
- For representing complex biomedical knowledge with intricate relationships
- When integrating diverse datasets from multiple sources
- In projects requiring automated reasoning and knowledge discovery
- For long-term collaborative efforts in knowledge representation
Case Studies: Taxonomy & Ontology in Action
Case Study 1: Gene Ontology (GO) The Gene Ontology (GO) project is a prominent example of ontology application in biomedical research. GO provides a comprehensive standardized vocabulary for describing gene and gene product attributes across species and databases. It consists of three interrelated ontologies:- Molecular function
- Biological process
- Cellular component
- Annotate genes and gene products with standardized terms
- Perform enrichment analyses to identify overrepresented biological processes in gene sets
- Integrate and compare genomic data across different species and experiments
- Anatomical main group
- Therapeutic subgroup
- Pharmacological subgroup
- Chemical subgroup
- Chemical substance
- Standardized drug classification across different countries and healthcare systems
- Efficient drug utilization studies and pharmacoepidemiological research
- Clear organization of pharmaceutical products for regulatory purposes
The Future of Data Organization in Biomedical Research
As biomedical research continues to generate vast amounts of complex data, the importance of effective data organization tools will only grow. Future developments in this field may include:- AI-driven ontology development - Leveraging artificial intelligence to assist in the creation and maintenance of large-scale biomedical ontologies
- Enhanced interoperability - Developing standards and tools to facilitate seamless integration between different ontologies and taxonomies
- Real-time knowledge graphs - Creating dynamic, self-updating knowledge representations that evolve with new scientific discoveries
- Personalized medicine applications - Utilizing ontologies to integrate diverse patient data for more precise diagnosis and treatment selection
Integration of Taxonomy & Ontology in Data Governance
While taxonomy and ontology serve different purposes, they’re not mutually exclusive. In fact, combining both approaches within a data governance framework can offer significant advantages. A well-defined taxonomy can provide the foundation for organizing data, while an ontology can overlay this structure with relationships and semantic connections, enabling more advanced data analysis and integration. Pharmaceutical and biotech companies increasingly rely on this integration to manage their vast data assets. For example, during drug development, taxonomies can organize preclinical and clinical data, while ontologies integrate this data with real-world evidence, such as electronic health records or genomic data, to identify new drug targets and predict adverse reactions. In the complex world of biomedical research and pharmaceutical development, both taxonomies and ontologies play vital roles in organizing and leveraging scientific knowledge. While taxonomies offer simplicity and ease of use for straightforward classification tasks, ontologies provide the depth and flexibility needed to represent intricate biomedical concepts and relationships. By understanding the strengths and limitations of each approach, researchers and data scientists can make informed decisions about how to structure their data effectively. As the life sciences continue to advance, the thoughtful application of these data organization techniques will be crucial in unlocking new insights, accelerating drug discovery, and ultimately improving patient outcomes. Are you ready to optimize your data organization strategy? Rancho Biosciences offers expert consultation and implementation services for both taxonomies and ontologies tailored to your specific research needs. Our team of experienced bioinformaticians and data scientists can help you navigate the complexities of biomedical data management, ensuring you leverage the most appropriate tools for your projects. Contact Rancho Biosciences today to schedule a consultation and discover how we can enhance your data organization capabilities, streamline your research processes, and accelerate your path to innovation, discovery, and scientific breakthroughs. Our bioinformatics services and expertise can propel your projects to new heights. Don’t miss the opportunity to take your data-driven endeavors to the next level.Unlocking the Secrets of Life: A Deep Dive into Single-Cell Bioinformatics
Miscellaneous
|Single-Cell Bioinformatics Defined
At its core, single-cell bioinformatics is a multidisciplinary field that combines biology, genomics, and computational analysis to investigate the molecular profiles of individual cells. Unlike conventional approaches that analyze a population of cells together, single-cell bioinformatics allows researchers to scrutinize the unique characteristics of each cell, offering unprecedented insights into cellular diversity, function, and behavior.The Power of Single-Cell Analysis
Unraveling Cellular Heterogeneity
One of the key advantages of single-cell bioinformatics is its ability to unveil the intricacies of cellular heterogeneity. In a population of seemingly identical cells, there can be subtle yet crucial differences at the molecular level. Single-cell analysis enables scientists to identify and characterize these variations, providing a more accurate representation of the true biological landscape.Mapping Cellular Trajectories
Single-cell bioinformatics goes beyond static snapshots of cells, allowing researchers to track and understand dynamic processes such as cell differentiation and development. By analyzing gene expression patterns over time, scientists can construct cellular trajectories, revealing the intricate paths cells take as they evolve and specialize.The Workflow of Single-Cell Bioinformatics
Cell Isolation & Preparation
The journey begins with the isolation of individual cells from a tissue or sample. Various techniques, including fluorescence-activated cell sorting (FACS) and microfluidics, are employed to isolate single cells while maintaining their viability. Once isolated, the cells undergo meticulous preparation to extract RNA, DNA, or proteins for downstream analysis.High-Throughput Sequencing
The extracted genetic material is subjected to high-throughput sequencing, generating vast amounts of data. This step is crucial for capturing the molecular profile of each cell accurately. Advances in sequencing technologies, such as single-cell RNA sequencing (scRNA-seq) and single-cell DNA sequencing (scDNA-seq), have played a pivotal role in the success of single-cell bioinformatics.Computational Analysis
The real power of single-cell bioinformatics lies in its computational prowess. Analyzing the massive datasets generated during sequencing requires sophisticated algorithms and bioinformatics tools. Researchers employ various techniques, including dimensionality reduction, clustering, and trajectory inference, to make sense of the complex molecular landscapes revealed by single-cell data.Applications Across Biology & Medicine
Advancing Cancer Research
Single-cell bioinformatics has revolutionized cancer research by providing a detailed understanding of tumor heterogeneity. This knowledge is crucial for developing targeted therapies tailored to the specific molecular profiles of individual cancer cells, ultimately improving treatment outcomes.Neuroscience Breakthroughs
In neuroscience, single-cell analysis has shed light on the complexity of the brain, unraveling the diversity of cell types and their functions. This knowledge is instrumental in deciphering neurological disorders and developing precise interventions.Precision Medicine & Therapeutics
The ability to analyze individual cells has immense implications for precision medicine. By considering the unique molecular characteristics of each patient’s cells, researchers can tailor treatments to maximize efficacy and minimize side effects.Challenges & Future Directions
While single-cell bioinformatics holds immense promise, it’s not without challenges. Technical complexities, cost considerations, and the need for standardized protocols are among the hurdles researchers face. However, ongoing advancements in technology and methodology are gradually overcoming these obstacles, making single-cell analysis more accessible and robust. Looking ahead, the future of single-cell bioinformatics holds exciting possibilities. Integrating multi-omics data, improving single-cell spatial profiling techniques, and enhancing computational tools will further elevate the precision and depth of our understanding of cellular biology. As we navigate the frontiers of biological research, single-cell bioinformatics stands out as a transformative force, unlocking the secrets encoded within the microscopic realms of individual cells. From personalized medicine to unraveling the complexities of diseases, the applications of single-cell analysis are vast and promising. As technology advances and researchers continue to refine their methods, the insights gained from single-cell bioinformatics will undoubtedly shape the future of biology and medicine, offering a clearer and more detailed picture of life at the cellular level. If you’re looking for a reliable and experienced partner to help you with your data science projects, look no further than Rancho BioSciences. We’re a global leader in data curation, analysis, and visualization for life sciences and healthcare. Our team of experts can handle any type of data, from NGS data analysis to genomics and clinical trials, and deliver high-quality results in a timely and cost-effective manner. Whether you need to clean, annotate, integrate, visualize, or interpret your data, Rancho BioSciences can provide you with customized solutions that meet your specific needs and goals. Contact us today to find out how we can help you with your data science challenges.Single-Cell Sequencing: Unlocking the Secrets of Cellular Heterogeneity in Biomedical Research
Miscellaneous
|Understanding Single-Cell Sequencing
Before exploring specific examples, it’s crucial to grasp the fundamental concept of single-cell sequencing. This advanced technique allows researchers to analyze the genetic material of individual cells, providing a high-resolution view of cellular diversity within a sample. Unlike traditional bulk sequencing methods, whose output is the average across many cells, single-cell sequencing provides the unique transcriptional profiles of each cell, offering invaluable insights into cellular function, developmental trajectories, and disease mechanisms.A Paradigm-Shifting Example: Single-Cell RNA Sequencing in Cancer Research
One of the most impactful applications of single-cell sequencing lies in the field of oncology. Let’s explore a groundbreaking example that demonstrates the power of this technology in revolutionizing cancer research and treatment strategies.The Study: Unraveling Intratumor Heterogeneity
In a landmark study published in Cell, researchers employed single-cell RNA sequencing (scRNA-seq) to investigate intratumor heterogeneity in glioblastoma, an aggressive form of brain cancer. This publication exemplifies how single-cell sequencing can provide crucial insights into tumor composition and evolution, with far-reaching implications for targeted therapies and personalized medicine.Methodology & Findings
The research team collected tumor samples from 13 patients diagnosed with glioblastoma. Using the 10x Genomics Chromium platform, they performed scRNA-seq on over 24,000 individual cells isolated from these tumors. This high-throughput approach allowed for an unprecedented level of resolution in analyzing the transcriptomes of cancer cells and the tumor microenvironment (TME). Key findings from this study include:- Cellular diversity - The researchers identified multiple distinct cell populations within each tumor, including malignant cells, immune cells, and stromal cells. This heterogeneity was previously undetectable using bulk sequencing methods.
- Transcriptional programs - By analyzing gene expression patterns at the single-cell level, the team uncovered specific transcriptional programs associated with different cellular states, including proliferation, invasion, and stemness.
- Tumor evolution - The study revealed insights into the evolutionary trajectories of glioblastoma cells, identifying potential mechanisms of treatment resistance and tumor recurrence.
- Immune microenvironment - Single-cell sequencing allowed for a detailed characterization of the tumor immune microenvironment, providing valuable information for developing immunotherapy strategies.
Implications for Drug Discovery & Personalized Medicine
This example of single-cell sequencing in glioblastoma research demonstrates the transformative potential of this technology for the pharmaceutical and biotech industries:- Target identification - By uncovering specific cellular subpopulations and the associated molecular signatures that set them apart, single-cell sequencing enables the identification of novel therapeutic targets for drug development.
- Precision medicine - The ability to characterize individual tumors at the cellular level supports the development of personalized treatment strategies tailored to each patient’s unique tumor composition.
- Drug resistance mechanisms - Single-cell analysis provides insights into the mechanisms of drug resistance, allowing researchers to design more effective combination therapies and overcome treatment challenges.
- Biomarker discovery - The high-resolution data obtained from single-cell sequencing facilitates the identification of biomarkers for early disease detection, treatment response prediction, and patient stratification.
Expanding Horizons: Single Cell-Sequencing across Biomedical Research
While the glioblastoma study serves as a powerful example, the applications of single-cell sequencing extend far beyond cancer research. Let’s explore how this technology is transforming other areas of biomedical research and drug development.Immunology & Autoimmune Disorders
Single-cell sequencing has revolutionized our understanding of the immune system’s complexity. For instance, one study published in the journal Nature Immunology used scRNA-seq analysis to characterize the heterogeneity of immune cells in rheumatoid arthritis patients, revealing novel cellular subtypes and potential therapeutic targets. This approach is enabling the development of more targeted immunotherapies and personalized treatments for autoimmune disorders.Neurodegenerative Diseases
In the field of neuroscience, single-cell sequencing is unraveling the intricate cellular landscape of the brain. Researchers have used this technology to create comprehensive atlases of brain cell types and identify specific neuronal populations affected in diseases like Alzheimer’s and Parkinson’s. These insights are crucial for developing targeted therapies and early diagnostic tools for neurodegenerative disorders.Developmental Biology & Regenerative Medicine
Single-cell sequencing is providing unprecedented insights into embryonic development and cellular differentiation. For example, a study published in Nature used scRNA-seq to map the entire process of embryonic organ development in mice. This knowledge is invaluable for advancing regenerative medicine approaches and developing stem cell-based therapies.Infectious Diseases
The COVID-19 pandemic has highlighted the importance of single-cell sequencing in understanding host–pathogen interactions. Researchers have used this technology to characterize the immune response to SARS-CoV-2 infection at the cellular level, informing vaccine development and identifying potential therapeutic targets.Challenges & Future Directions
While single-cell sequencing has undoubtedly transformed biomedical research, several challenges and opportunities remain:- Data analysis and integration - The sheer volume and ever-increasing complexity of single-cell sequencing datasets require sophisticated bioinformatics tools and analytical approaches. Developing robust algorithms for data integration and interpretation is crucial for maximizing the impact of this technology.
- Spatial context - Traditional single-cell sequencing methods lose information about the spatial organization of cells within tissues. Emerging spatial transcriptomics technologies aim to address this limitation, providing both transcriptional and spatial information.
- Multi-omics integration - Combining single-cell sequencing with other omics technologies, such as proteomics and epigenomics, will provide a more comprehensive understanding of cellular function and disease mechanisms.
- Clinical translation - While single-cell sequencing has shown immense potential in research settings, translating these insights into clinical applications remains a challenge. Developing standardized protocols and streamlined workflows for clinical implementation is essential for realizing the full potential of this technology in precision medicine.
Unraveling the DNA of Data: A Comprehensive Guide to Bioinformatics
Miscellaneous
|Defining Bioinformatics
At its core, bioinformatics is the application of computational tools and methods to collect, store, analyze, and disseminate biological data and information. It emerged as a distinct field in the 1970s but gained significant momentum with the advent of high-throughput sequencing technologies and the exponential growth of biological data in the 1990s and 2000s. Bioinformatics serves as a bridge between the vast amounts of raw biological data generated by modern laboratory techniques and the meaningful insights that can be derived from this data. It encompasses a wide range of activities, from the development of algorithms for analyzing DNA sequences to efficiently storing and retrieving complex biological information in databases.The Fundamental Components of Bioinformatics
Biological Data The foundation of bioinformatics is biological data. This includes:- Genomic sequences (DNA and RNA)
- Protein sequences and structures
- Gene expression data (RNA)
- Metabolomic data
- Phylogenetic information
- Sequence alignment algorithms (e.g., BLAST, Clustal)
- Gene prediction tools
- Protein structure prediction software
- Phylogenetic tree construction methods
- Variant calling methodologies/tools (e.g., Mutect2)
- Expression and transcriptomic analysis (e.g., DESeq2, edgeR, CellRanger, salmon)
- Machine learning and data mining techniques
- GenBank for nucleotide sequences
- UniProt for protein sequences and functional information
- PDB (Protein Data Bank) for 3D structures of proteins and nucleic acids
- KEGG for metabolic pathways
Key Applications of Bioinformatics
Transcriptomics, Genomics, & Proteomics The quantification and characterization of all aspects of the “central dogma” of biology (DNA->RNA->protein) is a critical component of bioinformatics analysis. Bioinformatics tools are essential for:- Assembling and annotating genomes
- Identifying genes and predicting their functions
- Comparing genomes across species
- Comparing genomic variants across populations and individual samples
- Analyzing protein sequences and structures
- Predicting protein-protein interactions
- Quantifying the amount of individual transcripts and/or proteins in an individual sample/cell
- Construct phylogenetic trees
- Identify conserved sequences across species
- Study the evolution of genes and genomes
- Analyze population genetics data
- Identifying potential drug targets and biomarkers
- Predicting drug-protein interactions
- Analyzing the results of high-throughput screening
- Designing personalized medicine approaches
- Analyzing individual genomes to identify disease risk factors
- Predicting drug responses based on genetic markers and other biomarkers
- Designing targeted therapies for cancer and other diseases
- Integrating diverse data types (genomic, proteomic, clinical) for comprehensive patient profiles
Challenges in Bioinformatics
While bioinformatics has made tremendous strides, it still faces several challenges. Data Deluge The exponential growth of biological data, particularly with the advent of next-generation sequencing technologies, poses significant challenges in data storage, processing, and analysis. Developing efficient algorithms, pipelines, and infrastructures to handle this data deluge is an ongoing effort in the field. Integration of Heterogeneous Data Biological systems are complex, and understanding them often requires integrating diverse data types (genomic, proteomic, metabolomic, clinical, etc.). Developing methods to effectively integrate and analyze these heterogeneous datasets together is a major focus of current bioinformatics research. Reproducibility & Standardization Ensuring the reproducibility of bioinformatics analyses and establishing standardized protocols for data generation and analysis are crucial challenges. Efforts are underway to develop best practices and standardized workflows to address these issues. Ethical & Privacy Concerns As bioinformatics deals with sensitive personal genetic information, addressing ethical concerns and ensuring data privacy are paramount. Developing secure data sharing protocols and establishing ethical guidelines for the use of genetic information are ongoing challenges in the field.Future Directions
Looking ahead, several exciting trends are shaping the future of bioinformatics. Machine Learning & Artificial Intelligence The integration of advanced machine learning techniques, including deep learning, is expected to revolutionize bioinformatics. These approaches can potentially uncover complex patterns in biological data that aren’t easily discernible through traditional methods. Single-Cell and Spatial Omics Advances in single-cell sequencing technologies are generating unprecedented insights into cellular and tissue heterogeneity. Bioinformatics will play a crucial role in analyzing and interpreting this high-resolution data. An extension of single cell analysis, spatial transcriptomics is rapidly advancing as well. With this technique, the transcriptomic profile of cells is calculated while leaving the tissue structure intact. This technique will rapidly advance our understanding of cellular signaling and how even the same cell types can differ depending on their physical context within a tissue. Multi-Omics Integration The integration of multiple omics data types (genomics, transcriptomics, proteomics, metabolomics) to gain a holistic understanding of biological systems is an emerging trend. Bioinformatics methods for multi-omics data integration and interpretation are actively being developed, requiring a deep understanding of the underlying data and analytical methods. Cloud Computing & Big Data Technologies The adoption of cloud computing platforms and big data technologies is enabling bioinformaticians to process and analyze massive datasets more efficiently. This trend is likely to continue, making bioinformatics tools and resources more accessible to researchers worldwide. As we look to the future, the field of bioinformatics is poised for even greater advances. With the continuing explosion of biological data and the development of more sophisticated computational tools, bioinformatics will undoubtedly play an increasingly vital role in shaping our understanding of biology and driving innovations in healthcare and biotechnology. For students, researchers, and professionals alike, gaining proficiency in bioinformatics is becoming essential for staying at the forefront of biological and biomedical research. As we continue to unlock the secrets encoded in our genes and proteins, bioinformatics will remain an indispensable tool in our quest to understand and harness the complexity of life. If you’re eager to harness the power of comprehensive data management in the life sciences and unlock new possibilities for your research or healthcare initiatives, look no further than Rancho BioSciences. Our bioinformatics services and expertise can propel your projects to new heights. Don't miss the opportunity to take your data-driven endeavors to the next level. Contact Rancho BioSciences today and embark on a journey of innovation and discovery.Harnessing the Power of Data Mining in Artificial Intelligence
Miscellaneous
|Data Mining Defined
Data mining is the process of discovering patterns, correlations, and useful information from large volumes of data. It combines techniques from statistics, machine learning, and database systems to analyze datasets and extract knowledge that can be used for decision-making, prediction, and understanding complex phenomena. In the context of AI, data mining goes beyond simple data analysis. It employs advanced algorithms and machine learning models, including large language models (LLMs) and small language models (SLMs), to automate the discovery process, enabling organizations to uncover insights that might be impossible to detect through manual analysis or traditional statistical methods.The Data Mining Process
The data mining process typically involves several key steps:- Data collection
- Data cleaning
- Data transformation
- Data exploration
- Pattern evaluation
- Knowledge interpretation and presentation
The Role of AI & Machine Learning in Data Mining
Artificial intelligence enhances data mining by automating the process and improving the ability to handle vast datasets. AI-driven data mining uses machine learning algorithms, including LLMs and SLMs, to detect patterns, predict trends, and offer real-time insights without constant human intervention. Machine learning (ML), a subset of AI, is particularly crucial in data mining because it enables systems to learn from data and improve over time. ML algorithms use historical data to make predictions or classifications without explicit programming. For example:- Supervised learning – Trains an algorithm on labeled data
- Unsupervised learning – Uses unlabeled data to identify patterns
- Reinforcement learning – AI system learns by receiving feedback from its actions and adjusting its approach
Key Data Mining Techniques in AI
Data mining in AI employs a wide range of techniques, each suited for different types of data and analytical goals. Some of the most common techniques include:- Classification
- Clustering
- Association rule mining
- Regression analysis
- Anomaly detection
Key Applications of Data Mining for Biosciences & Healthcare
Data mining in AI has specific applications in healthcare and pharmaceutical research. Here are some key applications. Extracting Data from Electronic Health Records (EHRs) AI-powered data mining techniques, particularly natural language processing (NLP) models like LLMs and SLMs, can extract crucial relevant information from unstructured text in electronic health record (EHR) systems. This includes analyzing pathology reports and doctors’ notes to identify key medical findings, diagnoses, and treatment recommendations. By automating this process, healthcare providers can quickly access relevant patient information, leading to more efficient and accurate decision-making. Mining Research for the Newest Trends Data mining techniques can be applied to conference proceedings, presentation transcripts, and research papers to identify emerging trends in healthcare and pharmaceutical research. This competitive intelligence helps organizations stay at the forefront of scientific developments, informing strategic decisions in research and development. LLMs and SLMs can be particularly effective in summarizing and extracting key insights from large volumes of scientific literature. Building Automated Knowledge Graphs Data mining techniques can be used to construct automated knowledge graphs from disparate data sources in healthcare and pharmaceutical research. These knowledge graphs can integrate information from scientific literature, clinical trials, patient records, and molecular databases. By connecting related concepts and entities, knowledge graphs provide a comprehensive view of complex biomedical relationships, facilitating drug discovery, treatment optimization, and personalized medicine approaches.Challenges & Considerations in Data Mining
While data mining offers immense potential, it also comes with several challenges and ethical considerations:- Data privacy and security
- Data quality and bias
- Interpretability
- Scalability
- Ethical use of insights
How Large Language Models Are Revolutionizing Scientific Research
Miscellaneous
|What Are Large Language Models?
Large language models are a subset of artificial intelligence (AI) that utilize deep learning techniques to understand and generate human language. They’re built using vast datasets and complex neural networks that allow them to predict and produce coherent text based on the input they receive. The “large” in LLMs refers to both the size of the dataset they’re trained on and the number of parameters—variables the model adjusts during training to improve its accuracy. Key characteristics of LLMs include:- Massive scale – Trained on billions of parameters and enormous datasets
- Versatility – Can perform a wide range of language tasks
- Context understanding – Can grasp nuances and context in language
How Do They Work?
LLMs function by processing text data through layers of artificial neurons, where each layer captures different aspects of the language. For instance, earlier layers might focus on identifying individual words and their meanings, while later layers capture more complex structures like sentences and paragraphs. The training process involves adjusting millions (or even billions) of parameters to minimize errors in text prediction, enabling the model to generate more accurate and contextually relevant outputs.What Are the Applications of Large Language Models in Science?
The integration of LLMs into scientific research has opened up new avenues for exploration and innovation. Here are some key areas where LLMs are making a significant impact. Enhancing Literature Review & Knowledge Discovery One of the most time-consuming aspects of scientific research is conducting comprehensive literature reviews. LLMs can automate this process by quickly scanning vast amounts of scientific literature, summarizing key findings, and identifying relevant studies. This capability not only saves researchers time but also ensures they don’t overlook critical information. For example, a researcher studying climate change can use an LLM to review thousands of related papers, extracting pertinent data on temperature trends, greenhouse gas emissions, and mitigation strategies. The model can also identify emerging trends and gaps in the literature, guiding future research efforts. Improving Data Analysis & Interpretation Scientific data often comes in unstructured forms, such as raw text from experiments, field notes, or patient records. LLMs can process and analyze this unstructured data, converting it into structured formats that are easier to interpret. This capability is particularly valuable in fields like genomics, where large datasets of genetic sequences need to be analyzed to identify patterns and correlations. In medicine, LLMs can assist in analyzing patient records to identify potential risk factors for diseases or predict outcomes based on historical data. This can lead to more personalized treatment plans and better patient care. Generating Hypotheses LLMs can assist scientists in generating hypotheses by:- Analyzing existing research and proposing potential areas of investigation
- Identifying gaps in current knowledge
- Suggesting novel connections between seemingly unrelated concepts
- Translate natural language queries into formal database queries
- Provide explanations of scientific concepts in plain language
- Assist in the interpretation of results from scientific instruments
What Are Some of the Challenges & Ethical Considerations?
While LLMs offer numerous benefits, their use in science also raises important challenges and ethical considerations. These include concerns about bias, transparency, and the potential for misuse. Addressing Bias in LLMs LLMs are trained on large datasets that often include biased or unbalanced information, reflecting societal prejudices. If not carefully managed, these biases can influence the outputs generated by the models, leading to skewed or inaccurate results. In scientific research, where objectivity is paramount, the presence of bias in LLMs could compromise the integrity of findings. Researchers must take steps to mitigate bias by curating diverse and representative training datasets, employing bias detection algorithms, and continuously monitoring the performance of LLMs. Ensuring Transparency & Reproducibility The complexity of LLMs can make their inner workings opaque, leading to challenges in understanding how they arrive at certain conclusions. In science, transparency and reproducibility are critical for validating results. The “black-box” nature of LLMs poses a risk to these principles, as it can be difficult to trace the reasoning behind the model’s outputs. To address this, researchers are developing techniques for “interpretable AI” that aim to make the decision-making processes of LLMs more transparent. Additionally, providing detailed documentation of the models’ training data, algorithms, and methodologies can enhance reproducibility. Balancing Innovation with Ethical Use The powerful capabilities of LLMs also come with the potential for misuse. In science, this could include generating misleading information, fabricating data, or automating the production of low-quality research. Ensuring LLMs are used responsibly requires establishing ethical guidelines and oversight mechanisms. Researchers and institutions must work together to develop best practices for the ethical use of LLMs, ensuring these tools contribute positively to scientific progress while minimizing risks.What Is the Future of Large Language Models in Science?
As LLMs continue to evolve, their role in scientific research is likely to expand. Future models will be more powerful, accurate, and adaptable, enabling new discoveries and innovations across various fields. Some potential developments include:- Advanced multimodal models – Integrating LLMs with other AI technologies, such as computer vision and robotics, to create multimodal models capable of processing and analyzing diverse types of data, from text to images and beyond
- Personalized research assistants – Developing LLMs tailored to individual researchers or research teams, offering personalized support for literature review, data analysis, and hypothesis generation
- Real-time collaboration tools – Creating LLM-powered platforms that facilitate real-time collaboration among scientists, enabling them to share ideas, data, and findings more efficiently
Data Governance in Data Science: Ensuring Quality, Security, & Compliance
Miscellaneous
|Defining Data Governance
Data governance refers to the framework of policies and procedures that guide how data is managed within an organization. It involves defining who is responsible for data, how it should be used, and ensuring compliance with legal and regulatory requirements. In the realm of data science, data governance ensures data is reliable, secure, and used ethically.Key Components of Data Governance
Effective data governance involves several key components. Data Quality Management Data quality is paramount in data science. Governance frameworks establish processes to ensure data accuracy, completeness, consistency, and timeliness. This includes:- Data profiling and cleaning
- Metadata management
- Data lineage tracking
- Quality control measures
- Access control and authentication
- Encryption and data masking
- Compliance with data protection regulations (e.g., GDPR, CCPA)
- Regular security audits and risk assessments
- Data modeling and standardization
- Integration of disparate data sources
- Data storage and retrieval optimization
- Scalability and performance considerations
- Data acquisition and ingestion
- Processing and transformation
- Storage and archiving
- Data disposal and retention policies
- Adherence to industry-specific regulations
- Ethical guidelines for data collection and usage
- Transparency in data practices
- Regular compliance audits
Benefits of Data Governance in Data Science
Implementing robust data governance practices in data science offers several benefits:- Enhanced data quality and reliability – By establishing clear standards and processes, data governance ensures data scientists work with high-quality, reliable information. This leads to more accurate analyses and better decision-making.
- Improved data security and risk mitigation – Robust governance frameworks protect sensitive data from breaches and unauthorized access, reducing the risk of data loss and regulatory noncompliance.
- Increased operational efficiency – Standardized processes and clear roles and responsibilities streamline data management, reducing duplication of efforts and improving overall efficiency in data science projects.
- Better collaboration and data sharing – Data governance facilitates seamless collaboration between different teams and departments by establishing common data standards and protocols for data sharing.
- Enhanced regulatory compliance – A well-implemented governance strategy ensures data science practices align with relevant regulations, reducing the risk of legal issues and reputational damage.
Best Practices for Implementing Data Governance in Data Science
Establish Clear Roles & Responsibilities Define specific roles within the data governance framework, such as:- Chief Data Officer (CDO)
- Data stewards
- Data owners
- Data custodians
- Data quality criteria
- Security protocols
- Data access and usage guidelines
- Compliance requirements
- Data catalogs and metadata repositories
- Data quality and profiling tools
- Master data management (MDM) systems
- Data lineage and impact analysis tools
- Providing regular training and education on data governance principles
- Encouraging adherence to data policies and best practices
- Recognizing and rewarding good data stewardship
- Conduct regular audits and assessments
- Establish key performance indicators (KPIs) for data governance
- Gather feedback from stakeholders and adjust strategies accordingly
- Involve key stakeholders in governance planning
- Demonstrate the value of data governance through tangible business outcomes
- Regularly review and adjust governance strategies to support evolving business needs
Challenges of Data Governance
While the benefits of data governance are clear, organizations often face challenges in implementation:- Resistance to change – Employees may resist new governance policies and procedures, viewing them as obstacles to their work.
- Complexity of data environments – Modern data ecosystems are often complex, making it challenging to implement comprehensive governance across all data sources and systems.
- Balancing governance and innovation – Striking the right balance between maintaining control and allowing for the flexibility needed in data science innovation can be difficult.
- Resource constraints – Implementing robust data governance requires significant time, effort, and financial resources, which can be challenging for some organizations.
Future Trends in Data Governance
As data science continues to evolve, data governance will need to adapt to new trends and technologies:- Artificial intelligence and machine learning – AI and machine learning will play a growing role in automating data governance tasks and enhancing data quality management.
- Data privacy – With increasing concerns about data privacy, organizations will need to prioritize data governance practices that ensure compliance with evolving regulations.
- Decentralized data management – The rise of decentralized data management systems, such as blockchain, may impact traditional data governance approaches and require new strategies.
The AI Revolution in Life Sciences: Transforming Discovery & Patient Care
Miscellaneous
|Understanding AI in Life Sciences
Artificial intelligence in life sciences refers to the use of advanced computational algorithms and machine learning techniques to analyze complex biological data, make predictions, and automate various processes in research and healthcare. This technology is capable of processing vast amounts of information at incredible speeds, identifying patterns and insights human researchers might miss. AI in life sciences encompasses various subfields, including:- Machine learning (ML)
- Deep learning
- Natural language processing (NLP)
- Computer vision
- Generative AI
- Robotics
Drug Discovery & Development
One of the most significant impacts of AI in life sciences is in the realm of drug discovery and development. Traditional drug development processes are time-consuming and expensive, often taking more than a decade and billions of dollars to bring a new drug to market. AI is dramatically accelerating this process with:- Predictive modeling – AI models analyze vast datasets of chemical compounds and biological interactions to predict the efficacy and safety of potential new drugs. These predictions help scientists identify promising candidates much faster than traditional methods.
- Automated screening – High-throughput screening of compounds is now more efficient with AI. Machine learning algorithms can sift through massive libraries of chemical structures to identify those most likely to succeed in clinical trials.
- De novo drug design – AI is also facilitating the creation of entirely new drugs. Using generative models, AI can design novel compounds that have never been synthesized before, optimizing for desired biological effects.
- Target discovery – AI is revolutionizing the identification of new drug targets. By analyzing complex biological data, including genomics, proteomics, and metabolomics, AI algorithms can uncover previously unknown disease mechanisms and potential therapeutic targets. This approach helps researchers focus on the most promising biological pathways and proteins, significantly reducing the time and resources needed to find new drug targets.
Personalized Medicine
Personalized medicine aims to tailor medical treatment to individual patients based on their genetic makeup, lifestyle, and other factors. AI is playing a crucial role in making this vision a reality with:- Genomic analysis – AI algorithms analyze genomic data to identify mutations and variations linked to diseases. This analysis helps in understanding individual patient risks and developing targeted therapies.
- Predictive analytics – By integrating data from electronic health records (EHRs), wearable devices, and other sources, AI can predict disease onset and progression. This predictive power allows for early intervention and more effective treatment plans.
- Tailored treatment plans – AI helps in creating personalized treatment plans by considering a patient’s unique genetic profile, lifestyle, and response to previous treatments. This approach increases the likelihood of treatment success and minimizes adverse effects.
Diagnostics
AI’s ability to analyze complex datasets with high precision is transforming the field of diagnostics. Early and accurate diagnosis is crucial for effective treatment, and AI is making significant strides in these areas:- Medical imaging – AI algorithms excel in analyzing medical images such as X-rays, MRIs, and CT scans. They can detect anomalies and diseases with remarkable accuracy, often surpassing human radiologists in speed and precision.
- Pathology – In pathology, AI systems analyze tissue samples to identify cancerous cells and other abnormalities. This application not only speeds up diagnosis but also reduces the subjectivity and variability associated with human interpretation.
- Disease prediction – By analyzing patterns in medical data, AI can predict the likelihood of disease development. For example, AI models can assess a patient’s risk of developing conditions like diabetes or cardiovascular diseases based on their medical history and lifestyle factors.
Clinical Decision Support
AI is increasingly being used to support clinical decision-making, helping healthcare providers make more informed choices about patient care. By analyzing vast amounts of medical literature, clinical guidelines, and patient data, AI systems can:- Suggest appropriate treatment options
- Flag potential drug interactions
- Identify patients at high risk for complications
Patient Care
AI isn’t just transforming research and diagnostics but also enhancing patient care. From virtual assistants to advanced robotic surgery, AI technologies are making healthcare more efficient and personalized with:- Virtual health assistants – AI-powered chatbots and virtual assistants provide patients with instant access to medical information, appointment scheduling, and medication reminders. These tools improve patient engagement and adherence to treatment plans.
- Robotic surgery – AI is enabling more precise and minimally invasive surgical procedures. Robotic systems guided by AI offer enhanced dexterity and accuracy, leading to better surgical outcomes and faster patient recovery.
- Remote monitoring – Wearable devices and sensors, combined with AI, enable continuous monitoring of patients’ vital signs and health metrics. This real-time data allows for proactive management of chronic conditions and timely interventions.
Predictive Healthcare
The predictive capabilities of AI are being harnessed to anticipate health trends and outcomes at both individual and population levels. AI algorithms can:- Forecast disease outbreaks
- Predict patient readmission risks
- Identify individuals likely to develop chronic conditions
Challenges & Ethical Considerations
While the potential of AI in life sciences is immense, it’s not without challenges and ethical considerations:- Data privacy – The use of AI requires access to large datasets, raising concerns about patient privacy and data security. Ensuring robust data protection measures is essential to maintain trust and comply with regulations.
- Bias and fairness – AI systems can inherit biases from the data they’re trained on, leading to biased outcomes. It’s crucial to develop and implement algorithms that are fair and equitable to avoid perpetuating disparities in healthcare.
- Regulatory hurdles – The integration of AI into clinical practice requires navigating complex regulatory landscapes. Ensuring AI applications meet stringent safety and efficacy standards is critical for their adoption.
- Transparency and accountability – AI decision-making processes can be opaque, making it challenging to understand and trust their recommendations. Developing transparent and interpretable AI models is essential for their acceptance in healthcare.
The Future of AI in Life Sciences
As AI technologies continue to advance, we can expect even more transformative applications in life sciences:- Virtual clinical trials that reduce the need for large-scale human testing
- AI-designed proteins and genetic therapies
- Real-time health monitoring and intervention through wearable devices
- More accurate prediction of drug side effects and interactions
- Advanced biomarker discovery for early disease detection
Flow Cytometry: Unraveling Cellular Mysteries in Research & Medicine
Miscellaneous
|Understanding the Basics of Flow Cytometry
Before delving into its applications, let’s briefly explain how flow cytometry works. The process involves:- Sample preparation – Cells are suspended in a fluid and labeled with fluorescent markers.
- Flow chamber – The cell suspension is passed through a narrow channel, aligning cells in single file.
- Laser excitation – As cells pass through the laser beam, they scatter light and emit fluorescence.
- Detection – Optical detectors capture the scattered light and fluorescence signals.
- Data analysis – Specialized software processes the signals, providing detailed information about each cell.
Medical Research & Drug Discovery
Flow cytometry plays a crucial role in advancing medical research and drug discovery. Immunology Studies Flow cytometry is extensively used to analyze immune cell populations, their activation states, and cytokine production. This information is vital for understanding immune responses and developing new therapies for autoimmune diseases, allergies, and infections. Cancer Research In cancer studies, flow cytometry helps researchers identify and characterize tumor cells, assess their proliferation rates, and analyze their response to potential treatments. It’s particularly useful in studying rare cancer stem cells and developing targeted therapies. Stem Cell Research Scientists use flow cytometry to isolate and study stem cells, which are crucial for regenerative medicine. The technique helps researchers identify specific stem cell markers and monitor their differentiation into various cell types. Drug Screening & Development Flow cytometry accelerates drug discovery by enabling high-throughput screening of compounds. Researchers can quickly assess a drug’s effects on cellular processes, toxicity, and efficacy.Clinical Diagnostics & Patient Care
Flow cytometry has become an essential tool in clinical settings. Hematological Disorders Flow cytometry is widely used to diagnose and monitor blood disorders such as leukemia and lymphoma. Flow cytometry can identify abnormal cell populations and track treatment progress. HIV/AIDS Monitoring CD4+ T cell counts, crucial for monitoring HIV progression and treatment efficacy, are routinely measured using flow cytometry. Organ Transplantation The technique assesses organ compatibility and monitors post-transplant rejection by analyzing donor-specific antibodies and immune cell activation. Rare Disease Diagnosis Flow cytometry is valuable in diagnosing rare genetic disorders by detecting specific cellular abnormalities or protein deficiencies.Environmental & Industrial Applications
Beyond medicine, flow cytometry finds applications in various other fields. Water Quality Assessment Environmental scientists use flow cytometry to analyze microbial populations in water samples, monitor water quality, and detect contamination. Food & Beverage Industry The technique is employed to assess microbial content in food products and beverages, ensuring quality control and safety. Bioprocess Monitoring In biotechnology, flow cytometry optimizes fermentation processes by analyzing cell viability and productivity in bioreactors. Marine Biology Researchers use flow cytometry to study phytoplankton populations in oceans, providing insights into marine ecosystems and climate change impacts.Emerging Applications & Future Prospects
As technology advances, new applications for flow cytometry continue to emerge. Single-Cell Genomics Combining flow cytometry with next-generation sequencing allows for high-resolution analysis of gene expression in individual cells, revolutionizing our understanding of cellular heterogeneity. Extracellular Vesicle Analysis Flow cytometry is being adapted to study extracellular vesicles, such as exosomes, which play crucial roles in cell-to-cell communication and disease progression. Microfluidic-Based Cytometry Miniaturized flow cytometry systems are being developed, enabling point-of-care diagnostics and expanding the technique’s accessibility. Artificial Intelligence Integration Machine learning algorithms are enhancing flow cytometry data analysis, improving accuracy and revealing complex cellular patterns.Challenges & Limitations
While flow cytometry is a powerful tool, it’s important to acknowledge its limitations:- Sample preparation complexity
- Potential for cell damage during analysis
- High equipment and maintenance costs
- Need for skilled operators and data analysts
Understanding Data Curation: Real-World Examples & Best Practices
Miscellaneous
|The Importance of Data Curation
Before diving into examples, it’s essential to understand why data curation is crucial. In today’s data-driven world, organizations collect vast amounts of information from diverse sources. However, this raw data is often unstructured and inconsistent, making it challenging to derive meaningful insights. Data curation addresses these issues by:- Ensuring data quality – Curation processes clean and validate data, eliminating errors and inconsistencies.
- Leveraging data usability – Curation transformations make data ready for various purposes, such as analysis, decision-making, and data-driven applications.
- Enhancing accessibility – Well-curated data is easily searchable and retrievable, saving time and resources.
- Facilitating data integration – Curated data from different sources can be seamlessly integrated, providing a holistic view.
- Preserving data for future use – Proper curation ensures data longevity, allowing it to be reused and repurposed.
Data Curation in Healthcare
In the healthcare industry, data curation is vital for maintaining patient records, research data, and clinical trial information. Example: The UK Biobank is a large-scale biomedical database and research resource containing in-depth genetic and health information from half a million UK participants. Data curation in this context involves:- Data cleaning – Ensuring patient data is free of errors and inconsistencies
- Annotation – Adding metadata to describe the data accurately
- Harmonization – Creating and applying rules to align metadata from different healthcare sources or longitudinal data to ensure consistency of a patient’s medical records
- Standardization – Mapping data with inconsistent medical terminology to common ontology or dictionary to provide a comprehensive picture of a patient’s medical history
- Integration – Combining genetic data with health records to provide comprehensive insights
- Archiving – Storing data securely for long-term use by researchers worldwide
Data Curation in Academic Research
Academic institutions generate a vast amount of research data that needs to be curated for reproducibility and future studies. Example: The Inter-university Consortium for Political and Social Research (ICPSR) curates social science data to ensure it is accessible and usable for research and teaching.- Data cleaning – Standardizing data formats and correcting errors
- Metadata creation – Documenting data collection methods and variables
- Storage and preservation – Ensuring long-term access through robust archiving solutions
- Sharing and access – Providing easy access to datasets for the academic community
Data Curation in Environmental Science
Environmental data, such as climate records, biodiversity databases, and satellite imagery, require meticulous curation for effective environmental monitoring and analysis. Example: The Global Biodiversity Information Facility (GBIF) is an international network that provides open access to data about all types of life on Earth.- Data aggregation – Collecting data from multiple sources, including museums, universities, and government agencies
- Standardization – Harmonizing data formats and units for consistency
- Quality control – Validating and cleaning data to ensure accuracy
- Open access – Making data publicly available to researchers and policymakers
Data Curation in the Corporate Sector
Businesses leverage data curation to optimize operations, enhance customer experiences, and drive strategic decision-making. Example: E-commerce companies like Amazon curate customer data to personalize shopping experiences and improve service delivery.- Data cleaning – Removing duplicate records and correcting inaccuracies
- Segmentation – Categorizing customers based on behavior and preferences
- Integration – Combining data from different touchpoints (website, app, in-store) to create a unified customer profile
- Analytics and insights – Using curated data to generate actionable insights for marketing and product development
Best Practices for Effective Data Curation
To maximize the benefits of data curation, organizations should adopt the following best practices:- Establish clear policies – Define data governance policies that outline standards for data collection, processing, and management.
- Design standard operating procedures (SOPs) – Effectively design SOPs to perform data curation processes.
- Use automated tools – Leverage data curation tools and technologies to streamline processes such as data cleaning, standardization (e.g., mapping to ontologies), integration, and metadata creation.
- Maintain data documentation – Ensure comprehensive documentation for all datasets, including metadata, to facilitate understanding and reuse.
- Implement robust security measures – Protect curated data from unauthorized access and breaches through encryption and access controls.
- Promote interoperability – Use standardized data formats and protocols to ensure data can be easily shared and integrated across systems.
- Regularly update data – Continuously monitor and update datasets to maintain their relevance and accuracy.
- Engage stakeholders – Involve relevant stakeholders in the curation process to ensure data meets their needs and expectations.
Understanding CDISC Standards: An In-Depth Look at Different Types
Miscellaneous
|Study Data Tabulation Model (SDTM)
The Study Data Tabulation Model (SDTM) is the foundational standard, and perhaps the most widely recognized of all the CDISC standards. It defines a standard structure for clinical trial datasets that are to be submitted to regulatory authorities, such as the U.S. Food and Drug Administration (FDA) and the European Medicines Agency (EMA). SDTM consists of over 50 domains, each corresponding to different types of data collected in a clinical trial. These include, for example:- DM (Demographics) – Data on subject demographics, such as age, sex, and race
- AE (Adverse Events) – Information on adverse events experienced by subjects
- CM (Concomitant Medications) – Details about medications taken by subjects in conjunction with the study treatment
- VS (Vital Signs) – Vital signs measurements, such as blood pressure and temperature
Analysis Data Model (ADaM)
The Analysis Data Model (ADaM) focuses on the creation of datasets that are used for statistical analysis. Built on top of SDTM, which standardizes data collection, ADaM standardizes data analysis. The ADaM datasets are created from STDM, extending the primary results from STDM with calculations specified for the trial analysis. The most common calculation is the determination of how a specific measured characteristic of a trial participant changes over the course of the trial. The calculations are described in specific documents providing the required link between the original data in STDM format and the analysis data in the Adam datasets. Most ADaM datasets align with an SDTM domain. For example:- ADSL (Subject-Level Analysis Dataset) – Contains one record per subject, including demographic and treatment information
- ADAE (Adverse Event Analysis Dataset) – Designed for analyzing adverse event data
Standard for Exchange of Nonclinical Data (SEND)
The Standard for Exchange of Nonclinical Data (SEND) is largely overlapping with SDTM but applies to nonclinical studies. Nonclinical studies, such as animal safety and toxicology studies, are an essential part of the drug development process. The SEND set of standards extends SDTM to include domains specific to the results from these studies. SEND provides a standardized format for nonclinical study data. Some components unique to SEND include:- BW (Body Weights) – Body weight measurements of the test animals
- MA (Macroscopic Findings) – Data on visual observations of the test animals
- OM (Organ Measurements) – A component of pathology data used to assess safety
- SEND-DART (Developmental and Reproductive Toxicology) – A related set of standards specific to the study of the effects of drug candidates on developmental and reproductive health
Clinical Data Acquisition Standards Harmonization (CDASH)
Clinical Data Acquisition Standards Harmonization (CDASH) defines standards for data collection at the clinical trial site. CDASH ensures data collected during a clinical trial is standardized from the very beginning. CDASH specifies the standard data collection fields and formats that should be used in case report forms (CRFs). These include:- Demographics – Standard fields for collecting demographic data
- Adverse Events – Standardized fields for recording adverse events
- Concomitant Medications – Standard formats for capturing medication data
Controlled Terminology
Controlled Terminology refers to the standardized set of terms used across all CDISC standards. This includes predefined lists of allowable values for certain fields, ensuring consistency in data reporting. Controlled Terminology includes dictionaries for various data points, such as:- MedDRA (Medical Dictionary for Regulatory Activities) – Standardized medical terminology for adverse event reporting
- WHO Drug Dictionary – Standardized terms for drug names and classifications
Exploring the Types of Images Digital Pathology Can Reveal
Miscellaneous
|Whole Slide Images (WSI)
Whole slide imaging is the cornerstone of digital pathology. This process involves scanning entire glass slides to create high-resolution digital images that can be viewed, navigated, and analyzed on a computer screen. WSIs provide several benefits:- Comprehensive analysis – Every part of the slide can be examined in detail, ensuring no area is overlooked.
- Remote access – Pathologists can access slides from anywhere, facilitating telepathology and consultations with experts worldwide.
- Enhanced collaboration – Digital slides can be easily shared among colleagues, promoting collaborative diagnostics and research.
Cellular and Tissue Images
Digital pathology allows for the detailed examination of individual cells and tissues, providing insights into their morphology and structure. These images include:- Hematoxylin and eosin (H&E) stained images – These are the most common types of stained slides used in pathology. H&E staining highlights cellular and tissue structures, aiding in the diagnosis of various conditions, including cancers.
- Immunohistochemistry (IHC) images – IHC uses antibodies to detect specific antigens in cells, providing critical information about protein expression patterns. This is particularly useful in identifying types and origins of tumors.
- Fluorescent stained images – Fluorescent stains help pathologists visualize specific components within cells, such as DNA, RNA, and proteins. These images are invaluable in research settings for studying cellular functions and disease mechanisms.
3D Reconstruction Images
Advancements in digital pathology now allow for the creation of three-dimensional reconstructions from two-dimensional slide images. 3D imaging offers several advantages:- Spatial understanding – Pathologists can better understand the spatial relationships among different structures within a tissue.
- Detailed analysis – 3D images provide more detailed information about tissue architecture, which can be crucial in understanding complex diseases.
- Improved diagnostics – This technology can enhance the accuracy of diagnoses by revealing features that might be missed in traditional 2D images.
Dynamic and Interactive Images
Digital pathology isn’t limited to static images. Interactive and dynamic imaging techniques include:- Virtual microscopy – Users can zoom in and out and navigate through digital slides as if using a physical microscope. This enhances the learning experience for medical students and professionals.
- Time-lapse imaging – This involves capturing images at different time points to study dynamic processes, such as cell growth, division, and response to treatments.
- Multiplex imaging – This technique allows for the simultaneous visualization of multiple biomarkers in a single tissue section, providing comprehensive insights into disease pathology.
Computational and AI-Enhanced Images
Artificial intelligence and machine learning are transforming digital pathology by providing computationally enhanced images, which can significantly enhance the efficiency and accuracy of pathology workflows and bioinformatics services. These include:- Quantitative analysis – AI algorithms can quantify various parameters, such as cell count, tissue density, and biomarker expression levels, providing objective and reproducible data.
- Pattern recognition – AI can detect patterns and anomalies in images that might be subtle or missed by the human eye. This is particularly useful in screening for cancers and other diseases.
- Predictive modeling – AI can analyze image data to predict disease outcomes and responses to treatments, assisting in personalized medicine.
Special Stains and Techniques
In addition to traditional H&E and IHC staining, digital pathology supports a variety of special stains and techniques, such as:- Periodic acid-Schiff (PAS) staining – Used to detect polysaccharides and mucosubstances in tissues, aiding in the diagnosis of fungal infections and glycogen storage diseases
- Masson’s trichrome staining – Highlights collagen fibers in tissues, useful in evaluating fibrosis and other connective tissue disorders
- Silver staining – Commonly used to visualize nerve fibers, spirochetes, and reticular fibers in tissues
Data Mining vs. Knowledge Mining: Unraveling the Key Distinctions
Miscellaneous
|Data Mining vs Knowledge Mining
Data mining and knowledge mining aren’t competing approaches but complementary ones. By appreciating the differences between these two processes, organizations can not only understand the present landscape but also shape the future. Data mining is the process of discovering patterns, correlations, and anomalies within large data sets to predict outcomes. By using a combination of statistical analysis, machine learning algorithms, and database management systems, data mining extracts valuable information from raw data. This process is instrumental in various industries, including life sciences and healthcare.Key Components of Data Mining
- Data selection – Identifying the relevant data necessary for analysis, such as scRNA-seq analysis
- Data curation – Removing inconsistencies, duplicates, and errors from the data set to ensure accuracy and reliability
- Data transformation – Converting data into an appropriate format or structure for mining
- Data integration – Combining data from multiple sources into a cohesive dataset
- Data mining – Employing algorithms to identify patterns and relationships
- Pattern validation – Validating the identify patterns on additional datasets to ensure they’re valid and useful
- Knowledge representation – Presenting the discovered knowledge in an understandable and actionable form
Understanding Knowledge Mining
Knowledge mining, on the other hand, goes beyond data mining. While data mining can be seen as the “what” of the data, knowledge mining is the “why” behind it. It involves not only extracting information from data but also synthesizing and contextualizing this information to generate actionable insights and knowledge. This process often incorporates elements of artificial intelligence, natural language processing, and semantic technologies to create a deeper understanding of the data. Knowledge mining could start with results produced by data mining.Key Components of Knowledge Mining
- Data acquisition – Gathering data from various structured and unstructured sources
- Data processing – Cleaning and preparing data for analysis
- Text and semantic analysis – Utilizing natural language processing to understand and analyze text data
- Machine learning and AI – Applying advanced algorithms to learn from data and improve over time
- Knowledge synthesis – Integrating data from different domains to form comprehensive knowledge
- Contextualization – Adding context to the extracted information to make it meaningful and applicable
- Knowledge representation – Displaying the synthesized knowledge in formats that facilitate decision-making, such as dashboards, reports, or visualizations
Differences between Data Mining & Knowledge Mining
Scope & objectives
- Data mining – Focuses primarily on finding patterns and correlations within data sets. Its main objective is to extract valuable information that can be used for predictive analysis.
- Knowledge mining – Goes beyond mere information extraction by creating a comprehensive understanding and actionable knowledge. It seeks to provide context and relevance to the data, enabling more informed decision-making.
Techniques & tools
- Data mining – Utilizes statistical models, machine learning algorithms, and database management tools. Techniques such as clustering, classification, regression, and association rule learning are commonly employed.
- Knowledge mining – Incorporates advanced GenAI and machine learning techniques along with natural language processing (NLP) and semantic technologies. It often uses ontologies, knowledge graphs, and cognitive computing to achieve its goals.
Outcomes & applications
- Data mining – Produces patterns, trends, and predictions that can be used for business intelligence, customer segmentation, fraud detection, and market analysis.
- Knowledge mining – Delivers deeper insights, strategic intelligence, and comprehensive knowledge that can drive innovation, enhance decision-making, and create competitive advantages.
Practical Applications
Data mining
- Healthcare – Predicting and tracking the spread of infectious diseases, aiding in public health planning and response by analyzing patient records. Analyzing patient data to identify the most effective treatments for specific conditions, improving patient outcomes and reducing healthcare costs.
- Life sciences – By mining genetic data, researchers can identify genetic variants associated with specific diseases, helping in the development of personalized medicine.
Knowledge mining
- Healthcare – Knowledge mining techniques can analyze patient data to identify risk factors for diseases and predict outbreaks. This allows for early intervention and preventive measures.
- Life sciences – By integrating genomic data with clinical information scientists can develop personalized treatment plans for patients based on their genetic profiles. This can lead to more effective treatments with fewer side effects.
Challenges & Considerations
Despite their potential, there are ethical implications and technical hurdles to consider in both approaches.Ethical implications
- Privacy concerns – Handling sensitive data with care
- Bias in data – Ensuring algorithms are fair and unbiased
Technical hurdles
- Data quality – Requires high-quality, curated data
- Complexity of analysis – Needs advanced tools and expertise
How FAIR Data Principles Ensure Open & Reproducible Research in Life Sciences
Miscellaneous
|FAIR Data Principles Explained
FAIR is an acronym for Findable, Accessible, Interoperable, and Reusable. These principles were first proposed in a 2016 paper by a group of scientists aiming to promote data management and stewardship in a way that enhances data sharing and reuse (Wilkinson, Dumontier et al. 2016). They emphasize machine-actionability, meaning data should be structured in such a way that it can be automatically found, accessed, and used with minimal human intervention. This is particularly vital as technology, such as that used by bioscience professionals who provide flow cytometry services, continues to evolve with lightning speed.Findability: The First Pillar
Findability refers to the ease with which data can be discovered by both humans and computers. Key aspects include:- Unique identifiers – Assigning a globally unique and persistent identifier to each dataset
- Rich metadata – Describing data with comprehensive metadata that includes the identifier of the data it describes
- Searchable resources – Ensuring data and metadata are registered or indexed in a searchable resource
Accessibility: Ensuring Data Availability
Accessibility means that once data is found, it should be readily retrievable:- Standardized protocols – Using standardized protocols that are open and free to access data by their identifier
- Authentication and authorization – Where necessary, implementing procedures for authentication and authorization
- Persistent metadata – Keeping metadata accessible even if the data is no longer available
Interoperability: Facilitating Data Integration
Interoperability is the ability of data to be integrated with other data in formats that can be used with applications or workflows for analysis, storage, and processing:- Knowledge representation – Utilizing a formal, accessible, shared, and broadly applicable language for knowledge representation
- FAIR vocabularies – Employing vocabularies and ontologies that follow FAIR principles
- Qualified references – Including qualified references to other metadata and data
Reusability: Maximizing Data Value
Reusability ensures data can be reused effectively:- Well-described data – Providing a rich description of the data with accurate and relevant attributes
- Clear usage license – Releasing data with a clear and accessible data usage license
- Detailed provenance – Associating data with detailed provenance
- Community standards – Meeting domain-relevant community standards
Why the FAIR Data Principles Are Important in Life Sciences
Life sciences research involves complex datasets, often derived from experiments, clinical trials, or real world data. The FAIR principles are vital for several reasons:- Enhanced collaboration – FAIR data facilitates collaboration among researchers and institutions. When data is findable and accessible, it’s easier to share insights and resources.
- Improved reproducibility – Reproducibility is a cornerstone of scientific research. By adhering to the FAIR principles, researchers ensure their work can be validated and replicated by others.
- Accelerated innovation – FAIR data allows for the rapid exchange of information, fostering innovation. Scientists can build upon each other’s work without reinventing the wheel.
- Increased transparency – In a world where data-driven decisions are critical, transparency is key. The FAIR principles establish a foundation for open science, promoting trust in research findings.
Implementing FAIR Principles
Implementing the FAIR principles requires careful planning and consideration of the following:- Metadata and data standards – Establishing standards for metadata ensures data can be easily found and understood by others.
- Data licensing – Clear data licensing informs users about what they can and cannot do with the data.
- Data preservation – Ensuring data is stored in secure and sustainable ways so it remains accessible over time.
- Data stewardship – Assigning responsibility for data management to individuals or teams who can oversee the FAIR implementation.
Application of the FAIR Data Principles in Life Sciences
Applying the FAIR data principles in life sciences requires a combination of technical, organizational, and cultural changes. Here are some steps to get started:- Adopt a data management plan (DMP) – A DMP outlines how data will be handled throughout its lifecycle. It includes details on storage, sharing, and archiving practices.
- Use standardized metadata – Metadata provides context for your data, making it findable and reusable. Follow established metadata standards relevant to your field.
- Assign unique identifiers – Ensure your data has unique identifiers like DOIs. This practice not only makes data findable but also tracks its use and citation.
- Choose open and interoperable formats – Opt for data formats that are widely accepted and platform-independent. This choice promotes interoperability and ensures data can be reused across different systems.
- Establish clear access policies – Define who can access your data and under what conditions. Transparent access policies encourage proper data use while protecting sensitive information.
- Create comprehensive documentation – Documentation should be thorough and user-friendly. This includes details about how the data was collected, processed, and any relevant analysis methods.
- Promote a culture of data sharing – Encourage researchers to share their data and recognize those who do. A culture that values data sharing supports the FAIR principles and fosters collaboration.
A Guide to Creating a Data Ontology
Miscellaneous
|Understanding Data Ontology
At its core, a data ontology is a structured representation of concepts within a specific domain and the relationships between them. It serves as a blueprint, guiding how data is organized, accessed, and understood. By defining entities, attributes, and relationships, a data ontology provides a common language for stakeholders, easing communication and collaboration.The Importance of Data Ontology
A well-defined data ontology streamlines communication, facilitates data integration, and enhances data quality. It acts as a common language, fostering collaboration and ensuring consistency across diverse datasets. By establishing a shared understanding of the domain, a data ontology enables organizations to derive meaningful insights, make informed decisions, and drive innovation.Key Components of a Data Ontology
- Conceptualization – Begin by identifying the core concepts relevant to your domain. This involves understanding the entities, attributes, and relationships that define your data landscape. By conducting domain analysis and stakeholder interviews, you can uncover the fundamental concepts that underpin your ontology.
- Taxonomy development – Organize concepts into a hierarchical structure, establishing parent-child relationships that reflect their semantic hierarchy. This taxonomy provides a framework for categorizing and classifying data, facilitating navigation and retrieval.
- Relationship definition – Define the relationships between concepts, specifying how they interact and influence each other. This step elucidates the connections within the data ecosystem, enhancing its comprehensibility and utility. Whether representing hierarchical, associative, or part-whole relationships, clarity and precision are paramount in defining relationships.
- Attribute specification – Describe the properties or characteristics associated with each concept. Attributes define the unique features of entities and provide valuable context for interpreting data. By specifying attributes such as data type, range, and cardinality, you establish a comprehensive understanding of the data landscape.
- Constraints and rules – Establish constraints and rules governing the behavior of concepts and relationships. This ensures data integrity and coherence, preventing inconsistencies and errors. Whether enforcing cardinality constraints, domain restrictions, or integrity rules, explicit constraints contribute to the robustness of the ontology.
Best Practices for Creating a Data Ontology
- Collaborative approach – Involve stakeholders from diverse backgrounds to ensure the ontology reflects the collective understanding of the domain. By soliciting input from domain experts, data analysts, and end users, you can capture a comprehensive view of the domain and promote buy-in across the organization.
- Iterative refinement – Embrace an iterative approach, continuously refining the ontology based on feedback and evolving requirements. By soliciting feedback from stakeholders and incorporating lessons learned from implementation, you can iteratively enhance the ontology’s effectiveness and relevance.
- Reuse existing standards – Leverage existing ontologies and standards whenever possible to avoid reinventing the wheel and promote interoperability. Whether adopting industry standards, domain-specific ontologies, or community-developed vocabularies, reusing existing resources accelerates ontology development and fosters compatibility with existing systems.
- Documentation – Thoroughly document the ontology, including its rationale, design decisions, and usage guidelines. Clear documentation enhances usability and facilitates knowledge sharing. By documenting the ontology’s purpose, scope, and semantics, you empower users to effectively utilize and extend the ontology.
- Validation and testing – Validate the ontology against real-world data and use cases to ensure its effectiveness and correctness. By conducting validation tests, such as consistency checks, satisfiability tests, and domain-specific validation procedures, you verify the ontology’s accuracy and fitness for purpose.
Tools & Technologies for Ontology Development
- Semantic web technologies – RDF (Resource Description Framework), OWL (Web Ontology Language), and SPARQL (SPARQL Protocol and RDF Query Language) provide powerful tools for ontology modeling and querying. By leveraging these standards, you can represent, reason about, and query ontological knowledge in a standardized and interoperable manner.
- Ontology editors – Tools like Protege, TopBraid Composer, and OntoStudio offer intuitive interfaces for creating and managing ontologies. By providing features such as visualization, editing, and ontology debugging, these tools simplify the ontology development process and enhance productivity.
- Graph databases – Graph databases such as Neo4j and Amazon Neptune excel at representing and querying interconnected data, making them well suited for ontology storage and retrieval. By storing ontological knowledge as a graph structure, these databases enable efficient traversal and querying of complex relationships within the ontology.
Challenges & Considerations
- Semantic ambiguity – Addressing semantic ambiguity and reconciling conflicting interpretations can be challenging, requiring careful negotiation and consensus building. By fostering open communication and collaborative decision-making, you can navigate semantic ambiguity and establish shared semantics within the ontology.
- Maintenance overhead – Ontologies require ongoing maintenance to accommodate changes in the domain and evolving data requirements. Adequate resources and processes must be allocated to ensure sustainability. By establishing governance procedures, version control mechanisms, and ontology maintenance workflows, you can mitigate maintenance overhead and ensure the longevity of the ontology.
- Scalability – Ensuring the scalability of the ontology to handle growing volumes of data and evolving complexity is essential for long-term viability. By adopting scalable ontology modeling practices, such as modularization, abstraction, and lazy loading, you can manage ontology complexity and scale gracefully with evolving requirements.
- Interoperability – Harmonizing ontologies across disparate systems and domains is a complex endeavor, necessitating standardization efforts and interoperability protocols. By adhering to established ontology engineering principles, such as modularity, reusability, and alignment, you can promote ontology interoperability and facilitate seamless integration with external systems.
The Transformative Power of Single-Cell Data in Biological Discovery
Miscellaneous
|Applications in Biological Research
- Cellular Heterogeneity Unraveled
- Disease Insights Revealed
- Precision Medicine Advancements
Technological Innovations Driving Single Cell Analysis
- Single Cell Sequencing Platforms
- Computational Algorithms
- Integration with Multi-Omics Data
Challenges and Future Directions
- Data Integration Complexity
- Spatial Transcriptomics
The 3 Vital Stages of Data Curation
Miscellaneous
|Stage 1: Collection and Assessment
The journey of data curation begins with the collection and assessment of raw data from various sources. These sources may include databases, APIs, IoT sensors, social media platforms, and more. Data collection is often a complex undertaking, requiring careful consideration of factors such as data sources, formats, and quality. The key tasks in this stage include:- Identifying relevant data sources
- Ensuring the data is collected ethically and legally
- Performing initial quality checks
- Assessing the data for completeness, accuracy, and relevance
Stage 2: Cleaning and Transformation
Once data is collected, it often requires cleaning and transformation to enhance its quality and usability. This stage, known as data cleaning or data preprocessing, involves identifying and rectifying errors, handling missing or incomplete values, and standardizing data formats. Activities in this phase include:- Removing duplicates and correcting errors
- Standardizing data formats
- Organizing data into structured formats
- Annotating and labeling data for easier access and analysis
- Aligning terms to custom vocabularies or ontologies
Stage 3: Storage and Preservation
Once data is cleaned and transformed, it needs a secure and reliable storage solution. This final stage of data curation involves choosing appropriate storage systems, establishing data security measures, and implementing protocols for data backup and preservation. Key considerations in this stage are:- Ensuring compatibility with existing data systems
- Establishing protocols for data storage and backup
- Implementing data governance policies
- Preparing the data for analysis and application
The Importance of Data Curation
Effective data curation is critical for maximizing the value of data assets and driving informed decision-making. By meticulously managing data throughout its lifecycle, organizations can ensure its accuracy, reliability, and accessibility. This, in turn, enables them to derive meaningful insights, identify trends, and uncover opportunities for innovation and growth.Benefits of Data Curation
- Improved data quality – Curation enhances the quality and reliability of data, making it more trustworthy for analysis and decision-making.
- Enhanced data usability – Well-curated data is easier to access, understand, and analyze, leading to better insights and outcomes.
- Regulatory compliance – Proper data curation ensures compliance with data privacy regulations and industry standards, reducing the risk of legal issues.
- Cost savings – Investing in data curation up front can save costs associated with poor-quality data, such as erroneous decisions or failed projects.
Challenges of Data Curation
- Data volume and variety – Managing large volumes of diverse data can be challenging, requiring robust curation processes and tools.
- Data quality issues – Addressing data quality issues, such as errors and inconsistencies, requires time and resources.
- Data security concerns – Ensuring data security and privacy throughout the curation process is crucial to protect sensitive information.
- Evolving technology – Keeping pace with rapidly evolving technology and data formats requires continuous adaptation and learning.
Understanding the Biological Data Driving Bioinformatics
Abstract
|Types of Biological Data
Biological data can be classified into different types according to their level of organization, complexity, and format. Some of the most common types of biological data are:- Sequence data – This data represents the linear order of nucleotides in DNA or RNA molecules or amino acids in proteins. Sequence data can be used to study the structure, function, and evolution of genes and proteins as well as their interactions and regulation. It can be obtained with various techniques, such as DNA sequencing, RNA sequencing, and mass spectrometry.
- Structure data – This is data that represents the three-dimensional shape and arrangement of atoms or molecules in biological macromolecules, such as proteins, nucleic acids, or protein–nucleic acid complexes. It can be used to study the physical and chemical properties of biological macromolecules as well as their interactions and functions, and it can be obtained with techniques such as X-ray crystallography, nuclear magnetic resonance (NMR), and cryo-electron microscopy.
- Expression data – Expression data measures the amount or activity of genes or proteins in a given biological sample, such as a cell, tissue, or organism. This data can be utilized to examine the gene and protein expression patterns and profiles as well as their regulation and response to various stimuli or conditions. Expression data can be obtained through various methods, including microarrays, quantitative PCR, and proteomics.
- Interaction data – This data identifies and characterizes the physical or functional interactions between biological molecules, such as proteins, nucleic acids, metabolites, and drugs. The data can be employed to study the biological networks and pathways that mediate various biological processes and functions. A variety of techniques can be used to obtain interaction data, including yeast two-hybrid, co-immunoprecipitation, and affinity purification.
- Phenotype data – This is the data that describes the observable characteristics or traits of a biological entity, such as a cell, tissue, organism, or population. Phenotype data is useful for studying the effects of genetic or environmental factors on the morphology, physiology, behavior, or disease susceptibility of biological entities. Microscopy, imaging, and clinical tests are common techniques used to obtain this type of data.
Sources of Biological Data
Biological data can be obtained from various sources, such as:- Experimental data – This is the data generated from laboratory experiments or field studies designed and conducted by researchers to test a specific hypothesis or question. Experimental data can provide direct and reliable evidence for a biological phenomenon or mechanism, but it can also be limited by the availability of resources, time, and ethical constraints.
- Public data – This is data collected and shared by researchers or organizations through public databases or repositories that are accessible online. It can provide a large and diverse amount of information for a biological topic or problem, but it can also be heterogeneous, incomplete, or inconsistent in quality and format.
- Simulated data – This form of data is the product of computational models or simulations based on mathematical or statistical assumptions or rules. Simulated data can provide a theoretical or hypothetical scenario for a biological system or process, but it can also be inaccurate, unrealistic, or oversimplified.
Genomic Data: The Blueprint of Life
At the heart of bioinformatics lies genomic data—the complete set of genes within an organism’s DNA. This data provides a comprehensive blueprint of life, enabling scientists to understand the hereditary information passed from one generation to the next. Genomic data is instrumental in studying the structure, function, evolution, and regulation of genes, unraveling the secrets of our genetic code.Transcriptomic Data: Decoding Gene Expression
While genomic data reveals the genes present in an organism, transcriptomic data unveils how these genes are expressed. It represents the RNA transcripts produced by active genes, shedding light on the dynamic nature of cellular processes. Understanding transcriptomic data is crucial for deciphering the intricate mechanisms that govern various biological functions, helping researchers pinpoint when and where specific genes are active. By obtaining genome-wide transcriptome data from single cells using high-throughput sequencing (scRNA-seq), researchers are able to use scRNA-seq analysis to detect cell subpopulations within certain conditions or tissues.Proteomic Data: Unraveling the Protein Landscape
Proteomic data focuses on the study of proteins, the functional workhorses of cells. This data reveals the types, quantities, modifications, and interactions of proteins within a biological system. By analyzing proteomic data, scientists gain insights into the intricate networks that govern cellular activities. This is particularly valuable in understanding diseases, as aberrations in protein expression or function often underlie pathological conditions.Metabolomic Data: Tracing Metabolic Fingerprints
Metabolomic data provides a snapshot of the small molecules present in a biological system, offering a glimpse into the metabolic activities of cells. This data is crucial for understanding how organisms process nutrients, produce energy, and maintain homeostasis. Metabolomic analysis is especially valuable in studying diseases with metabolic components, such as diabetes or metabolic syndrome.Epigenomic Data: Uncovering the Marks on DNA
Epigenomic data explores the chemical modifications that influence gene expression without altering the underlying DNA sequence. These modifications, such as DNA methylation and histone acetylation, play a pivotal role in regulating various cellular processes. Examining epigenomic data allows researchers to unravel the intricate epigenetic landscape that influences development, aging, and disease.Structural Data: Peering into Molecular Architecture
To truly understand the intricacies of biological systems, scientists rely on structural data. This includes information about the three-dimensional shapes of molecules, such as proteins and nucleic acids. Structural data is essential for elucidating the molecular mechanisms underlying biological processes, facilitating the design of targeted drugs and therapies.Microbiome Data: Exploring the Bacterial Universe Within
The human body is home to trillions of microorganisms collectively known as the microbiome. Microbiome data involves the study of the genetic material of these microbes, providing insights into their diversity, abundance, and functional roles. Understanding the microbiome is crucial for comprehending its impact on human health, from digestion to immune function.Integrative Data: Connecting the Dots Across Domains
In the ever-expanding landscape of bioinformatics, the real power lies in integrating diverse datasets. Integrative data analysis involves combining information from genomics, transcriptomics, proteomics, and more to gain a holistic understanding of biological systems. This multidimensional approach enables researchers to unravel complex biological phenomena and identify novel connections.Harnessing the Power of Biological Data
In the era of precision medicine and personalized therapies, the significance of biological data in bioinformatics cannot be overstated. It serves as the compass guiding researchers through the intricate landscapes of genomics, proteomics, and beyond. As technology continues to advance, the wealth of biological data available will undoubtedly propel scientific discoveries, ushering in a new era of understanding and manipulating the very fabric of life. Decoding the language of biological data opens doors to transformative possibilities, promising a future where we can harness the power of life’s code for the betterment of humanity. If you’re eager to harness the power of comprehensive data management in the life sciences and unlock new possibilities for your research or healthcare initiatives, look no further than Rancho BioSciences. Our bioinformatics services and expertise can propel your projects to new heights. Don't miss the opportunity to take your data-driven endeavors to the next level. Contact Rancho BioSciences today and embark on a journey of innovation and discovery.Exciting News from Rancho BioSciences! We’re thrilled to announce our participation at The 19th Annual Huntington’s Disease Therapeutics Conference! Our team presented 3 groundbreaking posters, contributing to the vital discussions on drug discovery & development. Together, we’re pushing boundaries in Huntington’s Disease research!
Abstract
|Streamlining Research: The Power of CDISC in Data Standardization
Miscellaneous
|CDISC Standards Explained
CDISC standards are a set of rules and specifications that define how to structure, format, and label clinical data and metadata. CDISC standards cover the entire clinical research life cycle, from protocol design and data collection to data analysis and submission. CDISC standards can be grouped into two categories: content standards and data exchange standards. Content standards define what data elements are required or recommended for a given domain or purpose. For example, content standards may specify what variables and values should be included in an adverse events dataset or a clinical trial registry. Data exchange standards define how to represent and transfer data and metadata in a consistent and interoperable way. For example, data exchange standards may specify how to use XML or JSON to encode and exchange data and metadata. Some of the most widely used CDISC standards are:- Protocol Representation Model (PRM) – Defines the elements and structure of a clinical trial protocol
- Clinical Data Acquisition Standards Harmonization (CDASH) – Defines the best practices for collecting and organizing clinical data on case report forms (CRFs)
- Study Data Tabulation Model (SDTM) – Defines the structure and format of clinical data for submission to regulatory agencies
- Analysis Data Model (ADaM) – Defines the structure and format of analysis-ready clinical data for statistical analysis and reporting
- Define-XML – Defines the metadata and documentation of clinical data and analysis datasets in XML format
- Operational Data Model (ODM) – Defines the structure and format of clinical data and metadata for data exchange and integration
The Benefits of CDISC Standards
CDISC standards can bring a variety of benefits to your clinical research, such as:- Ensuring consistency in data collection – CDISC standards provide a common language for data collection, ensuring information is consistently captured across different studies. This standardization enhances data quality and reduces the likelihood of errors, creating a more robust foundation for research outcomes.
- Facilitating interoperability– Interoperability is a key challenge in the realm of clinical research. CDISC standards act as a bridge, enabling interoperability among different systems and stakeholders. This not only expedites data exchange but also facilitates collaborative efforts in multi-center trials.
- Accelerating time to insight – CDISC standards streamline the integration of diverse data sources, eliminating the need for time-consuming data mapping and transformation. This efficiency accelerates the time to insight, allowing researchers, such as those who rely on NGS data analysis, to focus more on analysis and interpretation, ultimately expediting the entire research process.
- Enhancing regulatory compliance – Compliance with regulatory requirements is a critical aspect of clinical research. CDISC standards provide a framework that aligns with regulatory expectations, making it easier for researchers to meet compliance standards. This not only ensures the credibility of the research but also expedites the approval process.
- Improving collaboration and communication – In a collaborative research environment where multiple stakeholders are involved, effective communication is paramount. CDISC standards facilitate clear communication by providing a standardized format for data exchange. This not only fosters collaboration but also minimizes misunderstandings and discrepancies.
- Enabling reusability of data – CDISC standards promote the creation of reusable datasets. Researchers can leverage existing standards, making it easier to share data across studies. This not only saves time but also encourages a more sustainable and cost-effective approach to data management.
- Enhancing data quality and confidence – One of the challenges in data management is ambiguity in data interpretation. CDISC standards address this issue by defining clear data structures and variables. This reduces the chances of misinterpretation, enhancing the overall quality and reliability of the data.
- Improving traceability – CDISC standards enhance traceability by providing a clear lineage of data from its origin to the final analysis. This transparency not only instills confidence in the research findings but also aids in auditing processes, contributing to the overall credibility of the research.
How Is Sequence Data Utilized?
Miscellaneous
|Sequence Data & Bioinformatics
Bioinformatics is the field of science that applies computational methods to biological data, such as DNA, RNA, or proteins. Sequence data is a major form of biological data, as DNA, RNA, and proteins are all sequences of nucleotides or amino acids. It’s used to answer key questions in biology and medicine, such as how sequence variation and cellular levels of RNA and proteins influence physiology and disease. These fundamental questions are addressed through bioinformatics tasks, such as sequence alignment, sequence search, sequence annotation, sequence prediction, and sequence quantification. Sequence Alignment Sequence alignment is the process of arranging two or more sequences to identify regions of similarity or difference. It can be used to measure the evolutionary distance, functional similarity, or structural similarity among sequences. It’s also a key step toward sequencing an individual’s entire genome and quantifying cellular levels of RNA and proteins, as raw sequence data typically comes in the form of fragments which much be mapped to a reference genome, transcriptome, or proteome. Sequence Search Biological function is dictated not just from the literal sequence of DNA, RNA, and proteins, but also from patterns within them. For instance, sequence patterns determine where proteins and small molecules bind to DNA and RNA and where proteins interact with each other. Sequence search attempts to find these patterns, including motifs, domains, and signatures, which improves our understanding of biological function and plays an important role in therapeutics and personalized medicine. Sequence Annotation Sequence annotation adds information and metadata to sequences, including names, descriptions, functions, and locations along a genome. This enriches the understanding and interpretation of sequences and provides useful and accessible information or resources. For instance, sequence annotation can be used to label genes, exons, introns, and promoters in a genome and provide their names, functions, and interactions, which is especially important for downstream analysis. Sequence Prediction Sequence prediction is the process of filling in missing pieces and inferring information about a sequence, such as its structure, function, or evolution. This can be used to complete or improve the knowledge and analysis of sequences and provide novel and valuable insights or hypotheses. For example, sequence prediction can be used to predict the secondary or tertiary structure of a protein, the function or activity of a gene, or the evolutionary origin or fate of a sequence. Sequence Quantification Sequence quantification attempts to determine the levels of sequences present in a biological sample, such as cells and tissues. It relies on upstream bioinformatics tasks, including alignment and annotation, for determining expression levels of specific genes and proteins, and is a critical step toward analysis and interpretation of sequence data.Challenges for Scientists
Bulk and single-cell RNA sequencing are among the most commonly utilized technologies for examining gene expression patterns, both at the population level and the single-cell level. The sheer size of datasets produced by these analyses poses computational challenges in data interpretation, often requiring proficiency in bioinformatic methods for effective data visualization. The constant evolution of sequencing techniques and statistical methods adds an extra element of complexity, often creating a bottleneck for scientists who are eager to delve into RNA-seq datasets but lack extensive coding knowledge to tackle a new software tool or programming language.SEQUIN: Empowering Scientists by Democratizing Data Analysis
In response to these challenges, Rancho BioSciences collaborated with the National Center for Advancing Translational Sciences (NCATS), specifically the Stem Cell Translation Laboratory (SCTL), to develop SEQUIN, a free web-based R/Shiny app designed to empower scientists without bioinformatics services. SEQUIN allows users to effortlessly load, analyze, and visualize bulk and single-cell RNA-seq datasets, facilitating rapid data exploration and interpretation. SEQUIN is designed to serve as a comprehensive tool for the swift, interactive, and user-friendly analysis of RNA sequencing data for single cells, model organisms, and tissues. The integrated functionalities of the app facilitate seamless processes such as data loading, visualization, dimensionality reduction, quality control, differential expression analysis, and gene set enrichment. A key feature of the app enables users to create tables and figures that are ready for publication. As a free resource that’s available to the public, SEQUIN empowers scientists employing interdisciplinary approaches to directly explore and present transcriptome data by leveraging state-of-the-art statistical methods. Consequently, SEQUIN plays a role in democratizing and enhancing the efficiency of probing biological inquiries using next-generation sequencing data at the single-cell resolution level. Rancho BioSciences boasts extensive expertise in delivering services related to RNA-seq data, encompassing transcriptomics analysis, scRNA-seq analysis, clustering, and differential gene expression (DEG) analysis. As part of our innovative Single Cell Data Science Consortium, we’ve established a Four-Tier Data Model tailored for RNA-seq data. Our team has successfully integrated hundreds of datasets, constituting millions of samples. Additionally, Rancho BioSciences has developed atlases organized by therapeutic area and has supported customers with large-scale dataset ingestion workflows. Furthermore, we offer the flexibility to install SEQUIN behind your firewall, allowing for local deployment to meet your specific requirements. If you’re looking for a reliable and experienced partner to help you with your data science projects, look no further than Rancho BioSciences. We’re a global leader in bioinformatics services, data curation, analysis, and visualization for life sciences and healthcare. Our team of experts can handle any type of data, from genomics to clinical trials, and deliver high-quality results in a timely and cost-effective manner. Whether you need to clean, annotate, integrate, visualize, or interpret your data, Rancho BioSciences can provide you with customized solutions that meet your specific needs and goals. Contact us today to learn how we can help you with your data science challenges.The Distinctions between Data Models & Data Ontologies
Miscellaneous
|What Is a Data Model?
A data model is a representation of the structure, format, and constraints of the data in a specific context or application. It defines what kind of data can be stored, how it can be stored, and how it can be manipulated. Data models can be expressed in various ways, such as diagrams, schemas, tables, or code. A data model is usually designed for a specific purpose or use case, such as a database, a software system, or a business process. A data model can be tailored to meet the requirements and expectations of the data’s users and stakeholders, and it can be validated and tested to ensure its quality and consistency. There are different types of data models, depending on the level of abstraction and detail. Some common types are:- Conceptual data model – A high-level overview of the main concepts and entities in a domain and their relationships. It doesn’t specify any technical details or implementation aspects.
- Logical data model – A more detailed and formal representation of the data elements and their properties. It contains the same concepts and relationships as the conceptual data model, but adds details such as data types, cardinality, keys, and constraints. It’s independent of any specific database system or technology.
- Physical data model – A specification of how the data in the logical data model is physically stored and accessed in a particular database system or technology. It includes aspects such as tables, columns, indexes, partitions, views, etc.
- Relational data model – A data model that organizes data into tables with rows and columns and defines the relationships between them using keys and foreign keys.
- XML data model – A data model that represents data as a hierarchical tree of elements, attributes, and text and defines the rules and syntax for the data using schemas and namespaces.
- JSON data model – A data model that represents data as a collection of name-value pairs, arrays, and objects and defines the data using a lightweight and human-readable format.
What Is an Ontology?
An ontology is a formal specification of the concepts, properties, and relationships that exist in a domain of interest. It defines the meaning and semantics of the data and the rules and logic that govern the data. Ontologies can be represented in various ways, such as graphs, languages, or frameworks. An ontology is usually designed to be independent of any specific system. It can capture the common and shared knowledge that exists in a domain and can be used by different kinds of applications or tasks. It can also be linked and aligned with other ontologies to create a network of knowledge. An ontology can be used to:- Provide a common vocabulary and framework for data sharing and integration across different sources and applications
- Enable reasoning and inference over data, such as discovering new facts, validating consistency, or answering queries
- Enhance data quality and usability by adding metadata, annotations, and context to data
- Support data analysis and visualization by enabling semantic queries, filters, and navigation
- FOAF (Friend of a Friend) – An ontology that describes the concepts and relationships related to people, such as name, gender, age, friend, colleague, etc.
- SKOS (Simple Knowledge Organization System) – An ontology that defines the concepts and relationships related to knowledge organization systems, such as thesauri, classifications, taxonomies, etc.
- GO (Gene Ontology) – An ontology that describes the attributes and functions of genes and gene products, such as biological processes, cellular components, molecular functions, etc.
How Do Data Models & Ontologies Differ?
Data models and ontologies are both ways of representing and organizing data, but they differ in several aspects, such as:- Scope – Data models are often application-specific, while ontologies are application-independent. A data model focuses on the data that’s relevant and useful for a particular context or purpose, while an ontology focuses on data from a specific domain or field.
- Expressiveness – Data models have less expressive power than ontologies. Data models can only describe the structure and format of the data, while ontologies can also describe the meaning and semantics of the data. While data models can only define the data elements and their relationships, ontologies can also define the data properties, constraints, rules, and logic.
- Reusability – Data models are less reusable than ontologies. A data model is usually designed for a specific application or system and may not be compatible or interoperable with other applications or systems. An ontology is designed to be reusable and can be linked and integrated with other ontologies to create a network of knowledge.
How Are Data Models & Ontologies Related?
Data models and ontologies are both ways of describing data in a domain or context, but they have different focuses and purposes. Data models focus on the structure and organization of data, while ontologies also include the meaning and semantics of data. Data models are more concerned with how data is stored and manipulated, while ontologies are more concerned with how data is understood and interpreted. Data models and ontologies aren’t mutually exclusive. They can complement each other and work together to provide a comprehensive description of data. For example:- A conceptual data model can be derived from or aligned with an ontology to ensure consistency and coherence among the concepts and entities in a domain.
- A logical or physical data model can be enriched with information from an ontology to add more semantics and metadata to the data elements and their properties.
- An ontology can be derived from or mapped to a logical or physical data model to extract the meaning and context of the data elements and their relationships.
Why Are Data Models & Ontologies Important?
Data models and ontologies are important for effective data management and analysis. They can:- Improve data quality and consistency by defining clear rules and standards for data creation, validation, transformation, and integration
- Enhance data interoperability and reuse by enabling common understanding and communication among different data sources and applications
- Facilitate data discovery and exploration by adding rich metadata, annotations, and context to data
- Support data-driven decision-making by enabling semantic queries, reasoning, inference, and visualization over data
Decoding Data: The Distinctions between Bioinformatics and Data Engineering
Miscellaneous
|Bioinformatics: The Genomic Symphony Conductor
Bioinformatics serves as the conductor of the genomic symphony, orchestrating the analysis and interpretation of biological data. At its core, bioinformatics integrates biology, computer science, and statistics to extract meaningful insights from vast datasets, especially those derived from genomics, proteomics, and other high-throughput technologies. Bioinformatics uses a variety of methods, such as:- Sequence alignment and comparison
- Phylogenetic analysis and evolutionary modeling
- Gene annotation and prediction
- Functional genomics and transcriptomics
- Proteomics and metabolomics
- Structural bioinformatics and molecular modeling
- Systems biology and network analysis
Data Engineering: Building the Infrastructure for Scientific Insights
While bioinformatics focuses on the analysis of biological data, data engineering lays the foundation for efficient data processing and storage. Data engineers design and implement the infrastructure necessary for storing, managing, and accessing vast datasets, ensuring scientists can work with data seamlessly. Data engineering uses a variety of tools and technologies, such as:- Database management systems (SQL, NoSQL, etc.)
- Data integration and transformation tools (ETL, ELT, etc.)
- Data storage and processing frameworks (Hadoop, Spark, etc.)
- Data warehousing and lake solutions (Snowflake, S3, etc.)
- Data quality and monitoring tools (Airflow, Datadog, etc.)
- Cloud computing platforms (AWS, Azure, GCP, etc.)
Divergent Skill Sets: Bioinformaticians vs. Data Engineers
Bioinformaticians: Masters of Algorithms Bioinformaticians require a deep understanding of biology coupled with expertise in algorithm development and statistical analysis. Proficiency in programming languages like Python and R is essential for implementing algorithms that sift through biological data to uncover meaningful patterns and associations. Data Engineers: Architects of Infrastructure Conversely, data engineers are architects of data infrastructure. Their skill set revolves around database management, data modeling, and proficiency in languages like SQL. They design and maintain the systems that enable seamless data flow, ensuring researchers have access to accurate and timely information.Collaboration at the Nexus: Bioinformatics Meets Data Engineering
Interdisciplinary Synergy While bioinformatics and data engineering have distinct roles, their synergy is crucial for advancing life sciences. Collaborative efforts between bioinformaticians and data engineers ensure the analytical tools used by researchers are supported by robust infrastructure, fostering a holistic approach to scientific inquiry. Data Security and Compliance In the life sciences, where data security and compliance are paramount, the collaboration between bioinformatics and data engineering becomes even more critical. Data engineers implement secure data storage solutions, ensuring sensitive information adheres to regulatory standards. Bioinformaticians can then focus on extracting insights without compromising data integrity.The Tapestry of Data Unveiled
In the intricate tapestry of life sciences, bioinformatics and data engineering are threads that, when woven together, create a comprehensive understanding of biological systems. While bioinformatics deciphers the genomic code, data engineering provides the infrastructure for this genomic revelation. Recognizing and appreciating the differences between these disciplines is essential for harnessing their combined potential to propel scientific discovery into uncharted territories. As the fields continue to evolve, the collaboration between bioinformatics and data engineering services will undoubtedly shape the future of life sciences, unraveling the secrets encoded within the vast biological data landscape. Rancho BioSciences can help you with all your data management and analysis needs. Our bioinformatics services and expertise can propel your projects to new heights. As a global leader in data curation, analysis, and visualization for life sciences and healthcare, we’re the experts you can rely on for expert biotech data solutions, bioinformatics services, data curation, AI/ML, flow cytometry services, and more. Don’t hesitate to reach out to us today and see how we can help you save lives through data.Digital Pathology 101: What Life Scientists Need to Know
Miscellaneous
|The Essence of Digital Pathology
At its core, digital pathology involves the digitization of traditional pathology practices. Traditionally, pathologists examine tissue slides under a microscope to diagnose diseases and abnormalities. With digital pathology, this process undergoes a paradigm shift as glass slides are transformed into high-resolution digital images. These digital slides can be viewed, managed, and analyzed using computer technology, fundamentally altering the way pathologists interact with patient samples.Main Components and Technologies
Digital pathology consists of four main components: image acquisition, image management, image analysis, and image communication.- Image acquisition – This is the process of creating digital slides from glass slides using a WSI scanner, a device that captures multiple images of a slide at different focal planes and stitches them together to form a single high-resolution image that can be viewed and manipulated on a computer screen.
- Image management – This involves storing, organizing, and retrieving digital slides using a software application that allows users to access, view, and manipulate digital slides on a computer or mobile device.
- Image analysis – Image analysis is the process of extracting and quantifying information from digital slides using algorithms or models to perform various tasks, such as segmentation, classification, detection, or prediction.
- Image communication – This is the process of sharing and exchanging digital slides and data using a network or a platform—a system that connects users and devices and enables the transmission and reception of digital slides and data.
Benefits of Digital Pathology
Digital pathology has many advantages for life sciences, such as:-
- Improving accuracy – Digital pathology can reduce human errors and biases in diagnosis and research. Image analysis can provide objective and consistent measurements and classifications that can enhance the quality and reliability of pathology data.
- Increasing efficiency – Digital pathology can save time and resources by eliminating the need for physical storage, transportation, and handling of slides. WSI can enable faster scanning and viewing of slides, while image analysis can automate tedious and repetitive tasks.
- Enhancing collaboration – Digital pathology can facilitate communication and collaboration among pathologists and other professionals across different locations and disciplines. WSI can enable remote consultation and education, while image analysis can enable data sharing and integration.
- Advancing innovation – Digital pathology can enable new discoveries and applications in life sciences. Image analysis can provide new insights and biomarkers that can improve diagnosis, prognosis, treatment, and prevention of diseases. WSI can enable new modalities and platforms for pathology education and training.
- Data integration and analysis – Digital pathology generates vast amounts of data that can be leveraged for research purposes. The integration of digital slides with other clinical and molecular data opens avenues for comprehensive analyses, contributing to a deeper understanding of diseases and potential treatment options. This data-driven approach accelerates research efforts, bringing us closer to breakthroughs in medical science.
- Educational innovation – In the realm of education, digital pathology offers a dynamic platform for training the next generation of pathologists. Digital slides can be shared across educational institutions, providing students with a diverse range of cases for learning and examination. This fosters a more interactive and engaging learning experience, preparing future pathologists for the evolving landscape of diagnostic medicine.
Overcoming Challenges and Ensuring Quality
Digital pathology also faces some challenges that need to be addressed, such as:-
- Standardization and regulation – As digital pathology becomes more prevalent, standardization and regulatory measures are crucial to ensure the quality and reliability of digital diagnostic practices. The development of industry standards and guidelines is essential to address concerns related to image quality, data security, and interoperability, fostering trust in the accuracy of digital diagnoses.
- Integration with existing systems – Efficient integration of digital pathology with existing laboratory information systems (LIS) and electronic health records (EHR) is imperative for seamless workflow integration. Overcoming technical challenges and ensuring compatibility will be key to the successful adoption and integration of digital solutions in pathology laboratories.
- Cultural issues – Digital pathology requires a change in the mindset and behavior of pathologists and other stakeholders who are used to traditional methods. These include issues such as training, education, adoption, acceptance, trust, ethics, and responsibility.
The Future of Diagnostics Unveiled
Digital pathology is a dynamic and evolving field with many potential future trends, such as:- Personalized medicine – Digital pathology aligns with the broader shift toward personalized medicine. By combining digital pathology data with molecular and genetic information, healthcare professionals can tailor treatment plans based on an individual’s unique characteristics. This precision approach holds the promise of more effective and targeted therapies, heralding a new era in patient care.
- Artificial intelligence – The integration of artificial intelligence (AI) in digital pathology is a frontier that holds immense potential. AI algorithms can analyze large datasets, identify patterns, and assist pathologists in making more informed diagnoses. As these AI tools continue to evolve, they have the potential to significantly enhance the efficiency and accuracy of pathology workflows and bioinformatics services.
- Democratization and globalization – Increasing and extending the availability of digital pathology can enable the dissemination and distribution of resources and services to various regions and sectors, especially those that are underserved or underdeveloped.
Embracing the Digital Pathway to Healthier Futures
Digital pathology isn’t just a technological evolution. It’s a revolution that’s reshaping the landscape of diagnostics in the life sciences. From precision diagnostics to collaborative research and educational innovation, the impact of this technology is far-reaching. As we navigate the challenges and embrace the opportunities presented by digital pathology, we pave the way for a future where diagnostics aren’t just accurate but also personalized, ushering in a new era of healthcare. If you’re looking for a reliable and experienced partner to help you with your data science projects, look no further than Rancho BioSciences. We’re a global leader in data curation, analysis, and visualization for life sciences and healthcare. Our team of experts can handle any type of data, from NGS data analysis to genomics and clinical trials, and deliver high-quality results in a timely and cost-effective manner. Whether you need to clean, annotate, integrate, visualize, or interpret your data, Rancho BioSciences can provide you with customized solutions that meet your specific needs and goals. Contact us today to find out how we can help you with your data science challenges.Navigating the Realm of Bioinformatics Services
Miscellaneous
|- Sequence Analysis
- DNA sequencing – Assisting in processing and analyzing DNA sequencing data, facilitating tasks such as variant calling, structural variant analysis, and identification of genetic mutations
- RNA sequencing – Analyzing transcriptomic data to understand gene expression patterns, alternative splicing events, and non-coding RNA functionalities
- Structural Bioinformatics
- Predicting protein structures – Aiding in predicting three-dimensional protein structures, offering insights into their functions and interactions
- Drug discovery – Contributing to drug discovery through the analysis of molecular structures, predicting drug-target interactions, and identifying potential candidates
- Comparative Genomics
- Evolutionary analysis – Allowing researchers to compare genomes across different species, identifying evolutionary relationships and conserved regions
- Phylogenetics – Assisting in constructing phylogenetic trees, revealing the relatedness of organisms and their evolutionary history
- Functional Annotation
- Gene ontology analysis – Categorizing genes based on their molecular functions, cellular components, and biological processes
- Pathway analysis – Essential for deciphering the functional implications of genetic and molecular changes by understanding biological pathways and networks
- Biomedical research
- Accelerating genomic research – Expediting the analysis of large-scale genomic datasets, facilitating breakthroughs in understanding genetic contributions to diseases
- Precision medicine
- Personalized treatment plans – Identifying genetic variations influencing individual responses to medications, paving the way for personalized treatment strategies
- Agricultural genomics
- Crop improvement – Contributing to the identification of genes associated with desirable traits in crops, enabling the development of more productive and resilient varieties
- Environmental microbiology
- Microbial community analysis – Enabling the understanding of microbial community diversity and functions, aiding environmental studies and conservation efforts
- Saving time and resources – Outsourcing bioinformatics tasks to experts can reduce the need for hiring, training, and maintaining in-house bioinformatics staff and infrastructure.
- Improving quality and reliability – Using bioinformatics services can ensure the use of state-of-the-art methods and tools that are validated and updated regularly.
- Enhancing collaboration and communication – Sharing bioinformatics results and insights with collaborators and stakeholders can facilitate data exchange and knowledge transfer.
- Advancing innovation and discovery – Applying bioinformatics services can enable new insights and hypotheses that can lead to novel discoveries and applications.
- Expertise – The level of knowledge and experience of the bioinformatics staff in your field of interest
- Quality – The standards and procedures for ensuring the accuracy, reproducibility, and security of the bioinformatics results
- Communication – The frequency and mode of interaction between you and the bioinformatics staff throughout the project
- Flexibility – The ability to customize the bioinformatics service according to your requirements and preferences
- Delivery – The format, content, and timing of the bioinformatics deliverables
- Support – The availability and responsiveness of the bioinformatics staff for providing feedback, troubleshooting, and follow-up
Navigating the Depths: Understanding the Goals of Knowledge Mining
Miscellaneous
|Understanding Knowledge Mining
Knowledge mining is the process of extracting valuable knowledge from vast datasets, both structured and unstructured. It involves utilizing advanced technologies such as artificial intelligence, machine learning, and natural language processing to make sense of the information deluge. The primary objectives of knowledge mining go beyond basic data retrieval. At its core, knowledge mining revolves around deriving meaningful insights and facilitating informed decision-making by establishing connections (references) between and among disjointed pieces of information.Uncovering Hidden Patterns & Trends
One of the key goals of knowledge mining is to reveal hidden patterns and trends within the data. By employing sophisticated algorithms, businesses can identify relationships that might otherwise remain obscured. This enables life sciences professionals to make data-driven decisions and predict potential target liabilities, toxicities, or even efficacies. The utilization of these algorithms allows researchers and scientists to identify correlations, anomalies, and recurring themes, providing valuable insights for accelerating discoveries and breakthroughs.Enhancing Decision-Making Processes
Knowledge mining aims to empower decision makers by providing them with actionable insights. By analyzing historical data and identifying relevant patterns, scientists are able to make decisions about which medicine to advance, the target to focus on, or new assay to implement. This not only enhances the decision-making process but also minimizes risks and enhances overall operational efficiency, which contributes to the smooth progression of life sciences projects.Improving Information Retrieval
Efficient information retrieval is paramount, especially in life sciences research. Knowledge mining creates robust systems that enable scientists and researchers to access relevant information swiftly. The implementation of advanced search algorithms ensures professionals can efficiently navigate through complex datasets, fostering a more agile and responsive research environment.Customizing User Experiences
In the realm of life sciences, customization is key. Knowledge mining facilitates the extraction of insights from diverse data sources, enabling the customization of research approaches. By tailoring experiments and methodologies based on individual project requirements, scientists can ensure a more effective approach to life sciences research.Enhancing Innovation
Innovation is the lifeblood of life sciences research. Knowledge mining acts as a catalyst by providing a deeper understanding of genomics, clinical data, and related processes. By identifying areas for improvement and innovation, organizations can stay at the forefront of life sciences research, fostering a culture of continuous advancement.Detecting Anomalies
In the intricate landscape of life sciences data, knowledge mining plays a crucial role in detecting anomalies that may impact research outcomes. By analyzing vast datasets in real time, organizations can proactively identify irregularities, ensuring the integrity of research findings and maintaining high standards in data quality. As we navigate the intricate landscapes of life sciences, knowledge mining at Rancho BioSciences emerges as a transformative force. Our team’s unparalleled knowledge mining abilities extend beyond data extraction to encompass the transformation of raw information into actionable insights, propelling advancements in genomics, clinical data, and other critical areas. Embracing these objectives positions Rancho BioSciences at the forefront of life sciences research, equipped with the insights needed to accelerate discoveries, foster innovation, and make lasting contributions to the field. To learn more about our vast array of services, from knowledge mining to flow cytometry analysis, call us today.FAIR Data: What It Is & Why It Matters
Miscellaneous
|FAIR Data Defined
FAIR represents a set of guiding principles aimed at maximizing the value of data. Developed by a group of international experts representing academia, industry, and publishing, FAIR data principles serve as a roadmap for data management, ensuring information is well organized and easy to discover, access, share, and reuse.Findability: Navigating the Data Maze
The first pillar of FAIR emphasizes the importance of making data findable by both humans and computer systems. In the vast ocean of digital information, finding relevant data can be akin to searching for a needle in a haystack. FAIR data principles advocate for the use of unique identifiers, metadata, and standardized vocabularies to enhance discoverability and ensuring data is indexed and searchable. This ensures researchers and analysts can efficiently locate the data they need, saving time and resources.Accessibility: Breaking Down Data Silos
Even the most valuable data is rendered useless if it remains inaccessible. Accessibility is the second pillar of FAIR, encouraging the removal of barriers that hinder data retrieval. This involves providing open access to data, eliminating restrictions, and employing clear and comprehensive access protocols. It also ensures data can be retrieved in a usable format. FAIR data promotes inclusivity, allowing a broader audience to benefit from shared information and fostering collaborative research efforts.Interoperability: Bridging the Data Divide
Interoperability, the third pillar of FAIR, addresses the challenge of integrating diverse datasets from various sources. In the absence of standardized formats and structures, data silos emerge, hindering cross-disciplinary collaboration. FAIR data principles advocate for the use of common data models and standards, as well as providing clear data interfaces and APIs to facilitate seamless integration. This interoperability ensures data can be combined and analyzed cohesively, unlocking new insights and promoting a holistic understanding of complex phenomena.Reusability: Maximizing the Life Span of Data
The fourth and final pillar of FAIR focuses on reusability, acknowledging that data shouldn’t have a single-use purpose. By ensuring data is well documented, including clear methodologies and contextual information, FAIR principles enable others to reuse the data in new analyses and studies. This not only maximizes the value of the original research but also promotes a sustainable approach to data management.Examples of FAIR Data
There are many examples of FAIR data initiatives and projects across different domains and disciplines. Here are some of them:- The European Open Science Cloud (EOSC) – a federated infrastructure that provides access to open and FAIR data and services for research and innovation in Europe.
- The Global Biodiversity Information Facility (GBIF) – an international network that provides access to biodiversity data from natural history collections, research projects, citizen science initiatives, and more.
- The Human Cell Atlas (HCA) – an international consortium that aims to create a comprehensive reference map of all human cells using single-cell technologies and FAIR data practices.
- The COVID-19 Data Portal – a platform that enables the sharing and analysis of COVID-19 related datasets from different sources and domains using FAIR data principles.
The Impact of FAIR Data on Scientific Research
FAIR data principles are transforming the landscape of scientific research. With increased findability, accessibility, interoperability, and reusability, researchers can build upon existing knowledge more efficiently. Collaboration among scientific communities is enhanced, leading to accelerated discoveries and breakthroughs. FAIR data principles also contribute to the reproducibility of research, a cornerstone of scientific integrity, by ensuring the data underpinning studies is transparent and accessible.FAIR Data in Business: A Competitive Edge
Beyond the realm of academia, businesses are recognizing the transformative power of FAIR data. In a data-driven economy, organizations that harness the full potential of their data gain a competitive edge. FAIR principles enable businesses to streamline their data management processes, break down internal data silos, and extract meaningful insights. This, in turn, enhances decision-making, drives innovation, and ultimately contributes to the bottom line.Future Challenges for FAIR Data
While the adoption of FAIR data principles is gaining momentum, challenges persist. Implementation can be resource intensive, requiring investment in infrastructure, training, and cultural shifts. Additionally, issues related to privacy, data security, and ethical considerations must be carefully navigated. As technology, such as that used by professionals who provide flow cytometry services, continues to evolve, the FAIR principles themselves may require updates to remain relevant and effective. In a world inundated with data, the importance of effective data management cannot be overstated. FAIR data principles provide a comprehensive framework for ensuring data isn’t only managed efficiently but also utilized to its full potential. Whether in scientific research, business analytics, or technological innovation, the adoption of FAIR principles marks a crucial step toward a future where knowledge is truly unlocked and accessible to all. Maximize the potential of comprehensive data management in life sciences and unlock new opportunities for your research or healthcare initiatives by exploring the transformative capabilities of Rancho BioSciences. Our bioinformatics services and expertise can propel your projects to unparalleled success. Take the opportunity to take your data-driven endeavors to the next level. Contact Rancho BioSciences today and embark on a journey of innovation and discovery.Unlocking the Secrets of Life: A Deep Dive into Single-Cell Bioinformatics
Miscellaneous
|Single-Cell Bioinformatics Defined
At its core, single-cell bioinformatics is a multidisciplinary field that combines biology, genomics, and computational analysis to investigate the molecular profiles of individual cells. Unlike conventional approaches that analyze a population of cells together, single-cell bioinformatics allows researchers to scrutinize the unique characteristics of each cell, offering unprecedented insights into cellular diversity, function, and behavior.The Power of Single-Cell Analysis
Unraveling Cellular Heterogeneity
One of the key advantages of single-cell bioinformatics is its ability to unveil the intricacies of cellular heterogeneity. In a population of seemingly identical cells, there can be subtle yet crucial differences at the molecular level. Single-cell analysis enables scientists to identify and characterize these variations, providing a more accurate representation of the true biological landscape.Mapping Cellular Trajectories
Single-cell bioinformatics goes beyond static snapshots of cells, allowing researchers to track and understand dynamic processes such as cell differentiation and development. By analyzing gene expression patterns over time, scientists can construct cellular trajectories, revealing the intricate paths cells take as they evolve and specialize.The Workflow of Single-Cell Bioinformatics
Cell Isolation & Preparation
The journey begins with the isolation of individual cells from a tissue or sample. Various techniques, including fluorescence-activated cell sorting (FACS) and microfluidics, are employed to isolate single cells while maintaining their viability. Once isolated, the cells undergo meticulous preparation to extract RNA, DNA, or proteins for downstream analysis.High-Throughput Sequencing
The extracted genetic material is subjected to high-throughput sequencing, generating vast amounts of data. This step is crucial for capturing the molecular profile of each cell accurately. Advances in sequencing technologies, such as single-cell RNA sequencing (scRNA-seq) and single-cell DNA sequencing (scDNA-seq), have played a pivotal role in the success of single-cell bioinformatics.Computational Analysis
The real power of single-cell bioinformatics lies in its computational prowess. Analyzing the massive datasets generated during sequencing requires sophisticated algorithms and bioinformatics tools. Researchers employ various techniques, including dimensionality reduction, clustering, and trajectory inference, to make sense of the complex molecular landscapes revealed by single-cell data.Applications Across Biology & Medicine
Advancing Cancer Research
Single-cell bioinformatics has revolutionized cancer research by providing a detailed understanding of tumor heterogeneity. This knowledge is crucial for developing targeted therapies tailored to the specific molecular profiles of individual cancer cells, ultimately improving treatment outcomes.Neuroscience Breakthroughs
In neuroscience, single-cell analysis has shed light on the complexity of the brain, unraveling the diversity of cell types and their functions. This knowledge is instrumental in deciphering neurological disorders and developing precise interventions.Precision Medicine & Therapeutics
The ability to analyze individual cells has immense implications for precision medicine. By considering the unique molecular characteristics of each patient’s cells, researchers can tailor treatments to maximize efficacy and minimize side effects.Challenges & Future Directions
While single-cell bioinformatics holds immense promise, it’s not without challenges. Technical complexities, cost considerations, and the need for standardized protocols are among the hurdles researchers face. However, ongoing advancements in technology and methodology are gradually overcoming these obstacles, making single-cell analysis more accessible and robust. Looking ahead, the future of single-cell bioinformatics holds exciting possibilities. Integrating multi-omics data, improving single-cell spatial profiling techniques, and enhancing computational tools will further elevate the precision and depth of our understanding of cellular biology. As we navigate the frontiers of biological research, single-cell bioinformatics stands out as a transformative force, unlocking the secrets encoded within the microscopic realms of individual cells. From personalized medicine to unraveling the complexities of diseases, the applications of single-cell analysis are vast and promising. As technology advances and researchers continue to refine their methods, the insights gained from single-cell bioinformatics will undoubtedly shape the future of biology and medicine, offering a clearer and more detailed picture of life at the cellular level. If you’re looking for a reliable and experienced partner to help you with your data science projects, look no further than Rancho BioSciences. We’re a global leader in data curation, analysis, and visualization for life sciences and healthcare. Our team of experts can handle any type of data, from NGS data analysis to genomics and clinical trials, and deliver high-quality results in a timely and cost-effective manner. Whether you need to clean, annotate, integrate, visualize, or interpret your data, Rancho BioSciences can provide you with customized solutions that meet your specific needs and goals. Contact us today to find out how we can help you with your data science challenges.How Data Management Enhances Life Sciences Research
Miscellaneous
|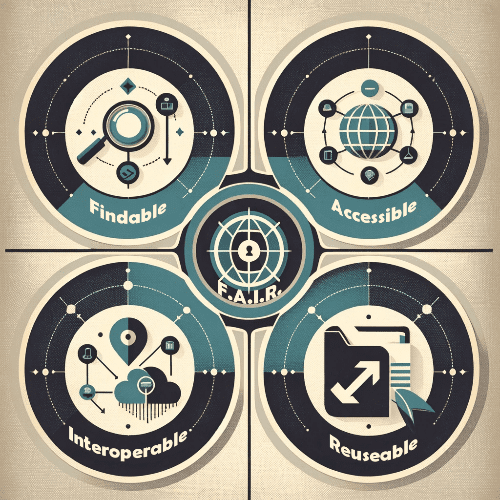
A Historical Perspective

Understanding Data Management in Life Sciences
Data management in the life sciences involves the collection, storage, organization, and analysis of data relevant to biological and biomedical research. It encompasses a wide range of data types, including genomics, proteomics, clinical data, imaging data, and more. The aim is to ensure this multi-modal data is effectively captured, securely stored, and readily accessible for analysis, thereby facilitating scientific discoveries and advancements in healthcare.Guiding Clients Through Their Data Management Journey
⦁ For Startups and Growing Companies – In the early stages, we assist clients in transitioning from basic data storage solutions to more sophisticated data management systems. Our expertise in effective data management helps these organizations unlock actionable insights, vital for their growth and development. ⦁ Scaling Up with AI-Driven Data Automation – As our clients expand, we introduce AI-driven automation to streamline their data processing and analysis. This transition is crucial for handling the increasing volume and complexity of data, turning it into a valuable asset for their operations. ⦁ Establishing a Robust Data Fabric for Global Entities – For clients with a global presence, we focus on building a seamless data fabric. This ensures data accessibility and integrity across different geographic locations and regulatory environments, supporting their international operations. ⦁ Empowering Research with Knowledge Mining – At every step, we delve into knowledge mining to bolster our clients’ research and development initiatives. Our services enable them to make informed decisions based on comprehensive and insightful data analysis.The Data Deluge
The life sciences are in the midst of a data deluge. Rapid advancements in technologies like DNA sequencing, mass spectrometry, and imaging have enabled researchers to generate vast amounts of data. Genomic sequencing is a prime example of the data-intensive nature of modern life sciences. A single experiment in this field can generate terabytes of data, presenting a substantial challenge in data management. Effectively handling this vast influx of data necessitates specialized approaches and talented experts to ensure accuracy, efficiency, and utility of the data.Challenges in Data Management
There are several significant challenges in the field of data management in life sciences: ⦁ Data acquisition – Obtaining data from various sources, such as experiments, observations, simulations, literature, or databases, can be challenging due to the diversity, complexity, and volume of data as well as the need for standardization and validation of data formats and protocols. ⦁ Data curation – Enhancing, annotating, and documenting data to make it more understandable and reusable can be challenging because of the dynamic and evolving nature of data along with the need for interoperability and integration of data across different domains and platforms. ⦁ Data storage – Preserving and securing data in appropriate locations and formats can be difficult due to the high cost and maintenance of data infrastructure and the need for scalability and reliability of data access and backup. ⦁ Data analysis – Applying statistical, computational, or visual methods to extract insights and knowledge from data is often challenging due to the heterogeneity, uncertainty, and noise of data as well as the need for innovation and collaboration of data methods and tools. ⦁ Data sharing – Disseminating and communicating data to relevant stakeholders, such as researchers, clinicians, regulators, or patients, can be a challenge because of the ethical, legal, and social implications of data disclosure as well as the need for transparency and reproducibility of data results. With regulations like GDPR and HIPAA, ensuring data privacy and compliance is a top priority in life sciences. To address these challenges and support data management in life sciences, there are various services and solutions available, such as: ⦁ Data governance frameworks – These are frameworks, such as data models, vocabularies/ontologies, and policies that provide guidelines for ensuring the quality, interoperability, security, privacy, and ethics of data. Data governance frameworks can help with tasks such as data ownership, stewardship, provenance, consent, and compliance. ⦁ Bioinformatics services – These are services that provide specialized expertise and resources for managing and analyzing biological data. ⦁ Bioinformatics services can help with tasks such as genome sequencing, gene expression analysis, protein structure prediction, phylogenetic analysis, and drug discovery. ⦁ Data repositories – These are the data hub repositories that provide online access and storage for curated and standardized data sets. Data repositories can help with tasks such as data discovery, retrieval, citation, and reuse. ⦁ Data management platforms – These are platforms that provide integrated software and hardware solutions for storing and processing large-scale data. Data management platforms can help with tasks such as data ingestion, transformation, querying, visualization, and mining.Tailoring Solutions for Pharma/Biotech
⦁ Research and Development (R&D) – In the R&D domain of Pharma/Biotech, our data management solutions are crafted to accelerate drug discovery and innovation. We focus on managing data from early-stage research, laboratory experiments, and preclinical studies. Our services ensure efficient handling of experimental data, fostering an environment where innovation thrives. ⦁ Manufacturing – In the manufacturing sector of Pharma/Biotech, our attention turns to managing data related to production processes, quality control, and supply chain logistics. We provide robust solutions to ensure data integrity and compliance with manufacturing standards, essential for maintaining product quality and safety. ⦁ Clinical Trials – For clinical trials, our data management approach is designed to handle the complexities of trial data. This includes patient data management, trial results, and regulatory compliance documentation. Our services support the efficient and secure management of clinical trial data, ensuring patient safety and the reliability of trial outcomes.Tailoring Solutions for Biobanks
⦁ Biobanking – Our data management solutions for biobanks emphasize the preservation, cataloging, and retrieval of biological samples. We focus on ensuring the integrity and traceability of data related to these samples. This supports the critical research activities in Pharma/Biotech, facilitating the accessibility of high-quality data for groundbreaking research and development.Benefits of Effective Data Management
Implementing effective data management offers numerous benefits: ⦁ Accelerated research – Researchers can access and analyze data more rapidly, expediting discoveries and drug development. ⦁ Enhanced collaboration – Well-organized data facilitates collaboration among research teams, even those working remotely or across borders. ⦁ Cost reduction – Efficient data management can reduce costs associated with data errors, redundancy, and inefficient use of resources. ⦁ Improved patient care – In healthcare, data management aids in personalized medicine by analyzing patient data to tailor treatments and interventions. ⦁ Scientific advancements – The foundation for new scientific knowledge and innovations is built on the robust management of data, allowing scientists to make breakthroughs in various life science fields. Data management in life sciences is at the heart of modern scientific research. It not only empowers researchers to make groundbreaking discoveries but also enhances healthcare and fosters collaboration in the global scientific community. As data continues to accumulate at an unprecedented pace, the role of data management remains central to unlocking the secrets of life’s most intricate processes. At Rancho Biosciences, our expedition in data management is about more than just navigating the complexities of data. It’s about empowering our clients to realize their goals in the life sciences sector. By providing bespoke data management solutions, we turn data into a strategic asset, driving innovation and accelerating scientific discoveries. We invite you to partner with us on this journey, harnessing our expertise to unlock the potential of your data and propel your projects to success. Don’t miss the opportunity to take your data-driven endeavors to the next level. Contact Rancho BioSciences today at (760) 642-6133 and embark on a journey of innovation and discovery.Unlocking the Secrets of Life: Bioinformatics & Its Applications
Miscellaneous
|Bioinformatics Defined
Bioinformatics is a multidisciplinary field that bridges biology, computer science, and mathematics. Its primary goal is to make sense of the massive volumes of biological data generated by modern scientific techniques. Researchers in this field use a combination of software, algorithms, and databases to store, analyze, and interpret biological data, from DNA sequences to protein structures.Genome Sequencing & Analysis
One of the most renowned applications of bioinformatics is genome sequencing and analysis. Scientists use advanced sequencing technologies to determine the order of nucleotides in DNA. Bioinformaticians then step in to process this vast amount of genetic information. By comparing and analyzing genomes, they can identify genes, regulatory elements, and mutations associated with various diseases, ultimately contributing to advancements in personalized medicine and genetic research.Proteomics & Structural Biology
Bioinformatics isn’t limited to genetics alone—it extends its reach to proteomics and structural biology as well. Proteomics aims to understand the functions and interactions of proteins within cells. By combining experimental data with computational methods, scientists can uncover protein-protein interactions and predict protein structures, with significant implications for understanding diseases. One of the main applications of proteomics is drug discovery, which is the process of finding new compounds that can modulate biological targets for therapeutic purposes. For example, bioinformatics can help to:- Discover new targets for drugs based on their role in disease pathways
- Screen potential drugs for their binding affinity and specificity to targets
- Design new drugs based on their structure and properties
- Test drugs for their efficacy and toxicity in cells and animals
Phylogenetics & Evolutionary Biology
Studying the evolutionary relationships between species is another crucial application of bioinformatics. Phylogenetics, the field devoted to this purpose, utilizes computational tools to analyze DNA, RNA, and protein sequences to construct evolutionary trees. This aids in deciphering the origin and diversification of species, tracking the spread of diseases, and understanding how life on Earth has evolved over billions of years.Metagenomics & Microbiome Studies
Bioinformatics not only analyzes data from humans and individual model organisms, but it also samples containing material from multiple species living together in a particular environment. Microbiomes and the bioinformatics subfield of metagenomics help us understand the role microorganisms play in various ecosystems. A microbiome is the collection of microorganisms that live in a particular environment, and they’re found all over our planet. From waterways and soil to our own bodies, the diversity and genetics of a given microbiome play an enormous role in how it interacts with and influences its environment. For example, the human gut and skin host microbiomes that are implicated in human health and disease. Bioinformaticians use metagenomics to study genetic material (like DNA) isolated from environments where microbiomes are found. Using current metagenomic methods, bioinformaticians can:- Quantitate the composition and genetic diversity of microbiomes from DNA collected from a particular body organ using techniques like DNA sequence alignment against curated databases of microbial genomes
- Assemble “metagenomes,” which are the predicted genomes of individual species, assembled from the pooled DNA of multiple species isolated from a biological environment
- Detect and monitor pathogens in water or soil samples
- Correlate microbiome makeup/genetics with clinical phenotypes
Single Cell Bioinformatics: A Game Changer
Single cell bioinformatics is a cutting-edge discipline within bioinformatics that’s revolutionizing our understanding of biology. Traditional methods often involved analyzing groups of cells together, masking the differences between individual cells. Single cell bioinformatics, however, zooms in to explore the unique characteristics of each cell.Unlocking Cellular Heterogeneity
One of the primary applications of single cell bioinformatics is to unravel the heterogeneity within tissues and organs. By analyzing individual cells, scientists can discover previously hidden variations, such as differences in gene expression, cell types, and cell states. This knowledge has profound implications for understanding development, disease progression, and treatment response.Studying Rare Cell Populations
Single cell bioinformatics is invaluable when studying rare cell populations within a sample. Traditional methods may miss these rare cells, but single cell analysis can pinpoint their presence and offer insights into their function. This is particularly useful in cancer research, where identifying and targeting rare cancer stem cells can be crucial for effective therapies.Personalized Medicine
As we delve into the era of personalized medicine, single cell bioinformatics plays a vital role. By understanding the unique characteristics of a patient’s cells, including those in tumors, researchers can tailor treatments to the individual, increasing the likelihood of success while minimizing side effects.Challenges & Future Prospects
While bioinformatics, including single cell bioinformatics, has achieved remarkable milestones, it still faces challenges. Handling and analyzing the vast amount of data generated in single cell studies requires robust computational infrastructure and expertise. Additionally, ethical considerations and data privacy are essential in this era of big data. As technology continues to advance, we can expect bioinformatics to evolve as well. The integration of artificial intelligence and machine learning will streamline data analysis, making it faster and more accurate. This will open new avenues for understanding complex biological systems and accelerate the development of novel treatments. Bioinformatics is a dynamic field with far-reaching applications in various areas of biology, from genetics to environmental science. Within this field, single cell bioinformatics stands out as a game changer, offering an unprecedented level of detail and insight into the intricacies of cellular biology. As we look to the future, bioinformatics holds the key to unlocking the secrets of life, driving progress in medicine, and enhancing our understanding of the natural world. Rancho BioSciences can help you with all your data management and analysis needs. Our bioinformatics services and expertise can propel your projects to new heights. As a global leader in data curation, analysis, and visualization for life sciences and healthcare, we’re the experts you can rely on for expert biotech data solutions, bioinformatics services, data curation, AI/ML, flow cytometry services, and more. Don't hesitate to reach out to us today at (760) 642-6133 and see how we can help you save lives through data.Rancho will be in Basel this week at BioTechX, booth# 806. Stop by to hear all about our brilliant services. Saving Lives Through Data!
Abstract
|Rancho Biosciences, the premier Data Science Services company headquartered in San Diego, California, is thrilled to announce its participation in Europe's largest biotechnology congress, BioTechX, a pivotal event that serves as a bridge between pharmaceutical companies, academia, and clinicians. The event aims to foster meaningful collaborations and catalyze innovation within the biotechnology and pharmaceutical industries.
As a leading player in the field of data science, Rancho Biosciences is dedicated to revolutionizing drug development and healthcare through the application of advanced technologies and data-driven strategies. The company is proud to spotlight its key service highlights at BioTechX, which include:
AI in Drug Development and Discovery: Rancho Biosciences harnesses the power of artificial intelligence to uncover groundbreaking insights and streamline the drug discovery process. They offer pre-packaged data sets specifically designed to train AI and machine learning algorithms.
Data Integration + FAIR: Rancho Biosciences goes beyond data management; they standardize and optimize data to be analysis-ready. Their commitment to the FAIR principles (Findable, Accessible, Interoperable, and Reusable) ensures data remains valuable and accessible. Their technology enables rapid data processing without compromising quality.
Bioinformatics R&D: With a team of world-class bioinformaticians, Rancho Biosciences brings extensive experience and domain knowledge to the table. They are dedicated to training and mentoring new talent for their clients.
Single Cell Genomics and NGS: Rancho Biosciences leads the way in curating single cell data sets, including deep annotations, and they have developed an SC Data Model to harmonize thousands of data sets. They also offer data sets with fewer metadata fields optimized for AI applications, all at competitive pricing.
Data Management, Storage, and Architecture: Learn how Rancho Biosciences can help organizations implement state-of-the-art infrastructure and strategies to manage large datasets effectively. Their services encompass building Data Lakes, knowledge portals, workflows, and more to meet the unique needs of their clients.
Digital Transformation: Rancho Biosciences isn't just observing the digital evolution of biotech; they are leading it. Attendees will discover how to harness the potential of digital tools and technologies to reshape and revolutionize the biotech landscape.
Real World Evidence: Rancho Biosciences' expertise in leveraging real-world data is changing the game by enhancing clinical outcomes and informing patient care and treatment methodologies.
Analytics Platforms: Explore the depths of data with Rancho Biosciences' robust analytics tools designed to decipher complex datasets and derive actionable insights for drug development.
About Rancho Biosciences:
Founded in 2012, Rancho Biosciences is a privately held company that offers comprehensive services for data curation, management, and analysis to organizations engaged in pharmaceutical research and development. Their client portfolio includes top 20 pharmaceutical and biotech companies, research foundations, government labs, and academic groups.
For further information and press inquiries, please contact:
Julie Bryant, CEO
Email: [email protected]
For more information about Rancho Biosciences and their participation in BioTechX, please visit www.RanchoBiosciences.com or stop by their booth# 806 at the event.
Source: Rancho BioSciences, LLC
Please visit Rancho next week at The Festival of Genomics, Booth# 4. Boston Convention & Expo Center, October 4 – 5.
Abstract
|Rancho Biosciences, the leading Data Science Service company, will be presenting expanded Data Science Services including our LLM work and a new product, Data Crawler that allows scientists to self-serve and find data sets they are looking for easily and quickly. The Festival of Genomics conference runs October 4-5, 2023, at the Boston Convention and Exhibition Center. Rancho’s mission of saving lives through data will be on full display through case studies, impact we have had on projects, biomarker discovery and clinical trials.
Julie Bryant, CEO and Founder, said: “Our goal is to be a partner and provide value through working efficiently with data, automating wherever possible and leveraging technologies such as AI/ML/NLP/LLM to provide high quality results with value and ROI.”
Rancho Biosciences is eager to introduce our cutting-edge services and insights to the attendees and engage with peers and pioneers alike to witness firsthand how we can turn your data into a catalyst for unmatched insights.
Why Visit Rancho Biosciences at The Festival?
Expert Insights: Delve deep into the realm of different data modalities with our scientists to better understand the latest breakthroughs and applications including single cell and spatial transcriptomics.
Tailored Solutions: Discover how our domain expertise and specialized offerings can pivot your genomics research or clinical endeavors to actionable results.
Interactive Discussions: Engage in meaningful conversations and solution-driven dialogues with our team of experts, discussing challenges and crafting a roadmap where we can build knowledge bases, data portals, unique analysis tools, workflows and pipelines.
About Rancho:
Founded in 2012, Rancho Biosciences is a privately held company offering services for data curation, management and analysis for companies engaged in pharmaceutical research and development. Its clients include top 20 pharma and biotech companies, research foundations, government labs and academic groups.
For press inquiries, please contact:
Julie Bryant
[email protected]
Rancho leverages the power of LLM (Large Language Models)
Abstract
|
At Rancho BioSciences, we leverage the power of large language models (LLMs) to provide a diverse range of services, enabling innovative ways to interact with data, including unstructured text, omics, and imaging data. Our expertise goes beyond the hype, delivering tangible value to our clients.
Our offerings include:
- Natural Language Processing: Gain actionable insights and enhance decision-making through advanced understanding and analysis of unstructured text data.
- Information Extraction: Streamline workflows and improve efficiency by accurately retrieving relevant information from vast data sources.
- Semantic Search: Enhance search functionality with context-aware results, ensuring accurate and relevant outcomes tailored to user intent.
- Prompt Engineering: Optimize communication and interaction with LLMs through expertly designed prompts that generate high-quality responses.
- Fine-tuning: Customize and adapt existing foundational models for seamless integration within the client's environment, maximizing performance and effectiveness.
In addition, we specialize in natural language querying (NLQ), making internal and public datasets easily accessible across large organizations. Our approach focuses on delivering tailored solutions that meet your unique requirements, driving tangible results and exceeding expectations.
RanchoBiosciences Offers CDISC-Compliant Data Curation Services Via SDTM
Abstract
|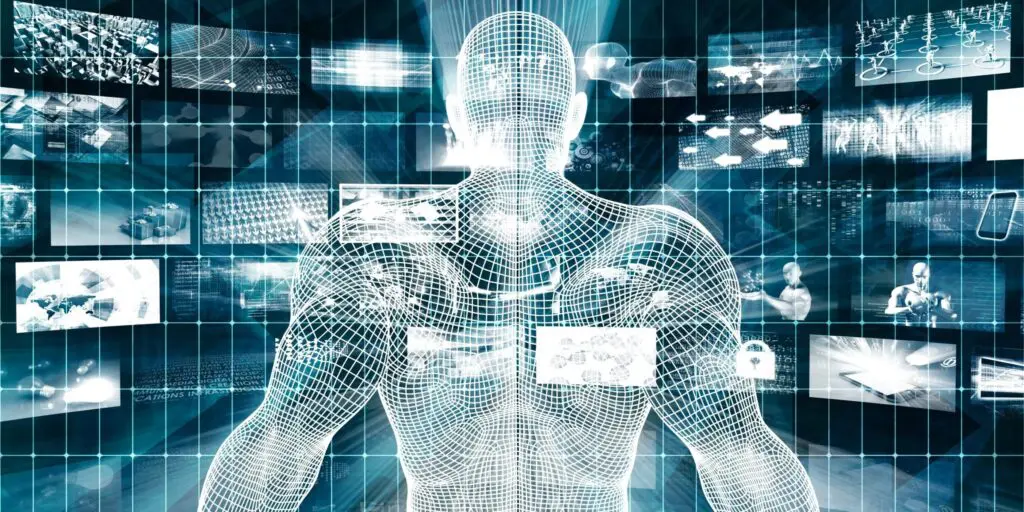
The Clinical Data Interchange Standards Consortium (CDISC) was developed to ensure healthcare, clinical, and medical research data are consistently presented and interoperable as a way of improving medical research. CDISC standards also help ensure data is FAIR (Findable, Accessible, Interoperable, and Reusable), which maximizes the data’s impact in terms of sharing capabilities, reducing R&D costs and timelines, and accelerating innovation.
The Study Data Tabulation Model (SDTM) is the CDISC-compliant standard format for data submitted to the FDA and other regulatory authorities. However, ensuring data adheres to the SDTM format can consume valuable time and resources, especially when data is derived from multiple studies.
Rancho BioSciences has developed a semi-automated workflow combining automated and manual curation, designed to flag and correct mistagged fields. This script, which first creates a preliminary tagged summary file, goes through a rigorous manual quality control protocol to ensure all domains, fields, and code lists are updated to current SDTM standards.
The resulting tagged summary file undergoes a final automated step, designed to eliminate unnecessary fields, reformat values to adhere to SDTM standards, and reorder columns per domain standards. Rancho BioSciences’ SDTM curation services create high-quality, accurate, and reliable data files to lead researchers towards actionable insights.
Rancho BioSciences is partnering with public and private research institutions
Abstract
|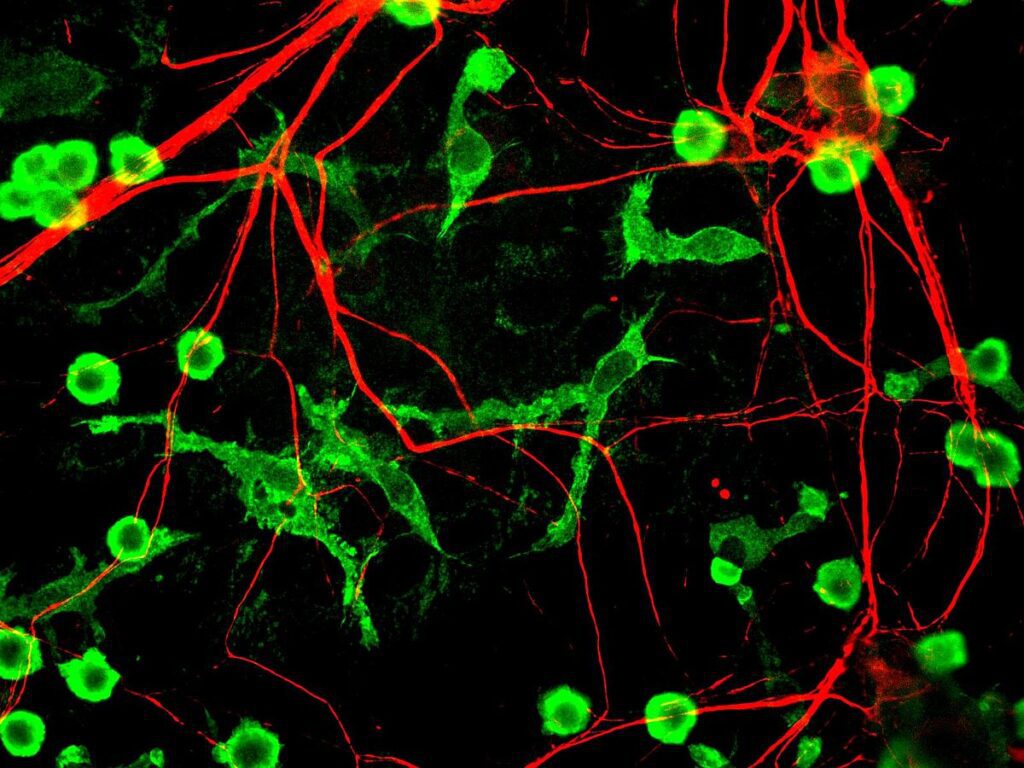
Rancho BioSciences is partnering with public and private research institutions to develop a comprehensive data catalog of transcriptomic studies of myeloid cells. These highly complex cells exhibit high plasticity and context-specific functions, making them difficult to study. Collecting and organizing data from existing transcriptomic studies will help researchers gain a global perspective on myeloid lineages and how they impact aging and disease.